Image segmentation is a key task in computer vision, often used in industries like medical imaging, autonomous driving, and more. Recent advancement in AI have led to the development of specialized tools that make image annotation and segmentation faster, easier, and more efficient. Here’s a rundown of some of the best image segmentation tools in 2024, detailing their strengths, tool-sets, and features for automatic segmentation and quality assurance.
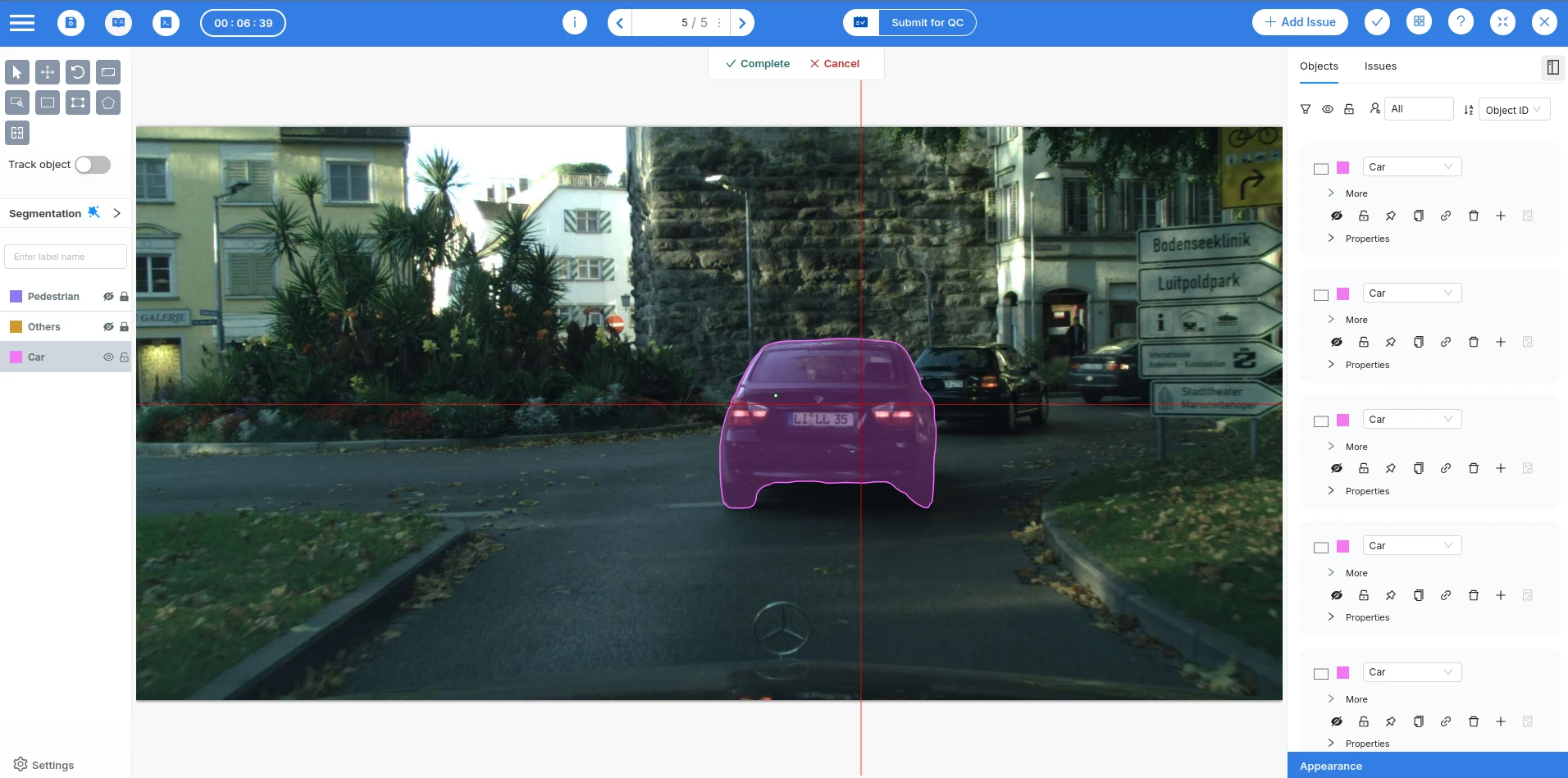
Mindkosh
Segmentation Tools: Mindkosh offers the polygon tool for quickly drawing object boundaries. The polygon tool along-with a robust layering system, automatic merging and snapping of neighboring objects, allow you to label images for segmentation extremely accurately.
Instance Segmentation Capabilities: Mindkosh supports labeling images with both semantic as well as instance masks, offering quick previews, flexible coloring, and easy exporting of the created segmentation masks.
Automatic Segmentation Features: Magic segment - Mindkosh's interactive segmentation tool can draw accurate object boundaries in just 2-3 clicks. Start with an initial boundary, and interact with the tool to refine the boundary further. Magic segment provides a more accurate boundary creation than the popular SAM segmentation model.
Quality Assessment and Assurance Features: Mindkosh allows you to create your own workflow and move data between Annotation, Validation, QC and complete modes. For performing QA, users can choose to setup honeypot or Inter annotator agreement on the platform itself, and view detailed analytics on quality metrics, time spent and number of objects labeled over time by each user.
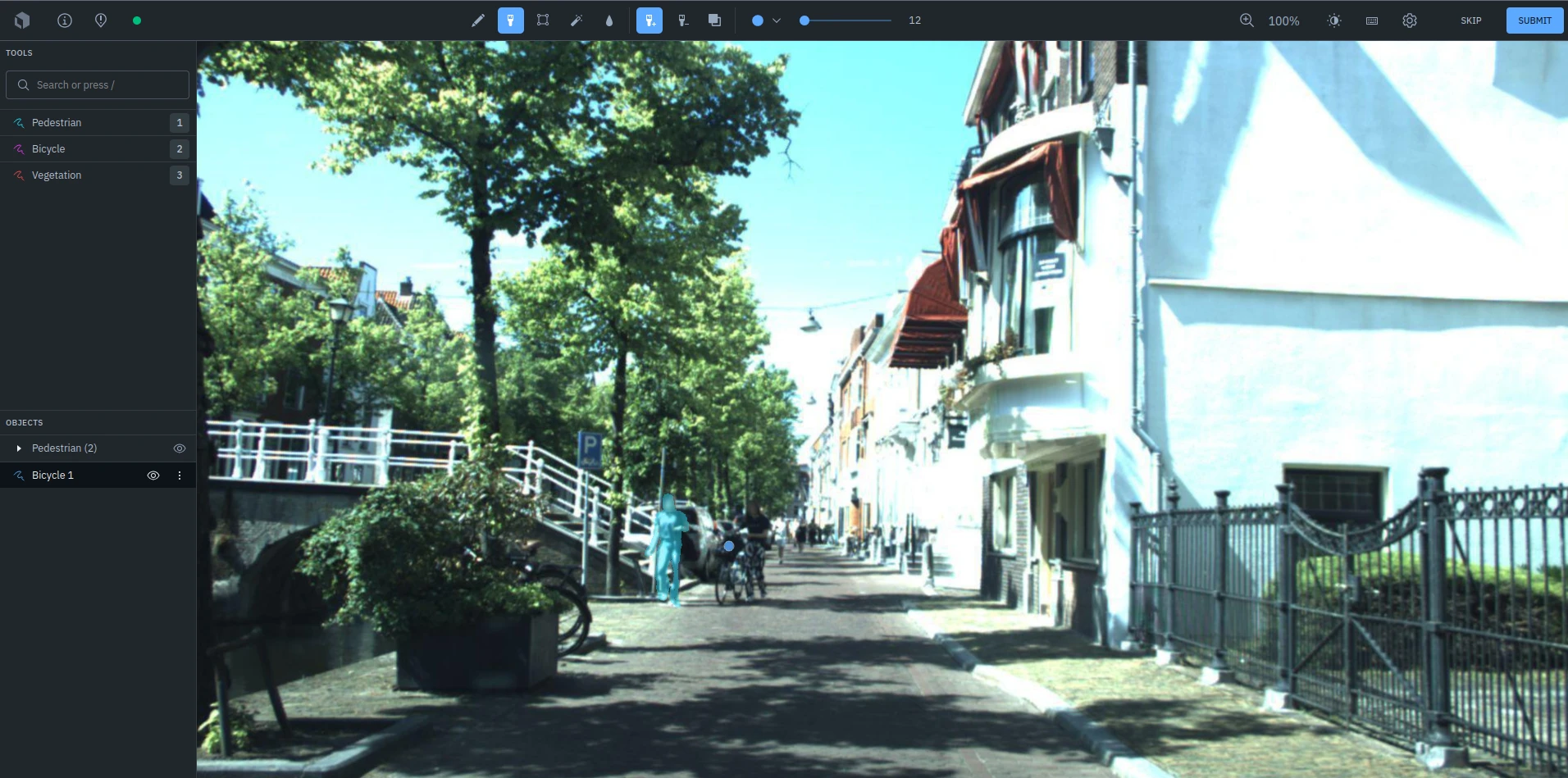
Labelbox
Labelbox is one of the first data-labeling platforms that supports a wide variety of tasks, including image segmentation, object detection, and classification. The platform is highly versatile and emphasizes collaboration, which is beneficial for teams working on large-scale labeling projects.
Segmentation Tools: Labelbox offers a comprehensive set of segmentation tools, including the polygon tool for precise boundary drawing, a brush tool for finer edits, and a pen tool for freehand drawing. This makes it flexible for both intricate segmentation and broader, simpler annotations.
Instance Segmentation Capabilities: Labelbox can handle instance segmentation, enabling the annotation of multiple instances of the same object within a single image, which is crucial for detailed labeling tasks in industries like medical imaging and autonomous driving.
Automatic Segmentation Features: Labelbox integrates AI-powered auto-segmentation features that reduce the manual effort required for annotating images. Its ML-assisted labeling helps speed up the process by predicting boundaries based on previous annotations, allowing for a semi-automatic workflow.
Quality Assessment and Assurance Features: Labelbox provides robust quality assurance tools. It allows teams to set up review workflows where annotations are reviewed by multiple team members before approval. The platform also supports model-assisted labeling that flags potential errors or inconsistencies in annotations, ensuring the final dataset meets quality standards.
V7 Darwin
V7 Darwin is a relatively new AI-powered platform focused on annotation and data labeling. It supports multiple use cases, from medical imaging to satellite imagery, and is optimized for AI-assisted workflows.
Segmentation Tools: V7 Darwin offers an intuitive set of tools such as brush, pen, and polygon tools for detailed segmentation. These tools provide precise control over annotations, making them ideal for segmenting complex objects.
Instance Segmentation Capabilities: V7 Darwin excels at instance segmentation by allowing users to easily mark and track multiple instances of the same class within an image, supporting advanced tasks like object tracking.
Automatic Segmentation Features: One of the standout features of V7 Darwin is its neural network-based auto-segmentation. The platform allows users to train models directly on the platform to automate the segmentation process, improving efficiency with AI-powered predictions that help guide manual annotations.
Quality Assessment and Assurance Features: V7 Darwin features QA workflows where every annotation goes through multiple review stages, ensuring high-quality datasets. Additionally, it provides inter-annotator agreement metrics that assess consistency between different annotators, which is essential for maintaining data integrity in large projects.
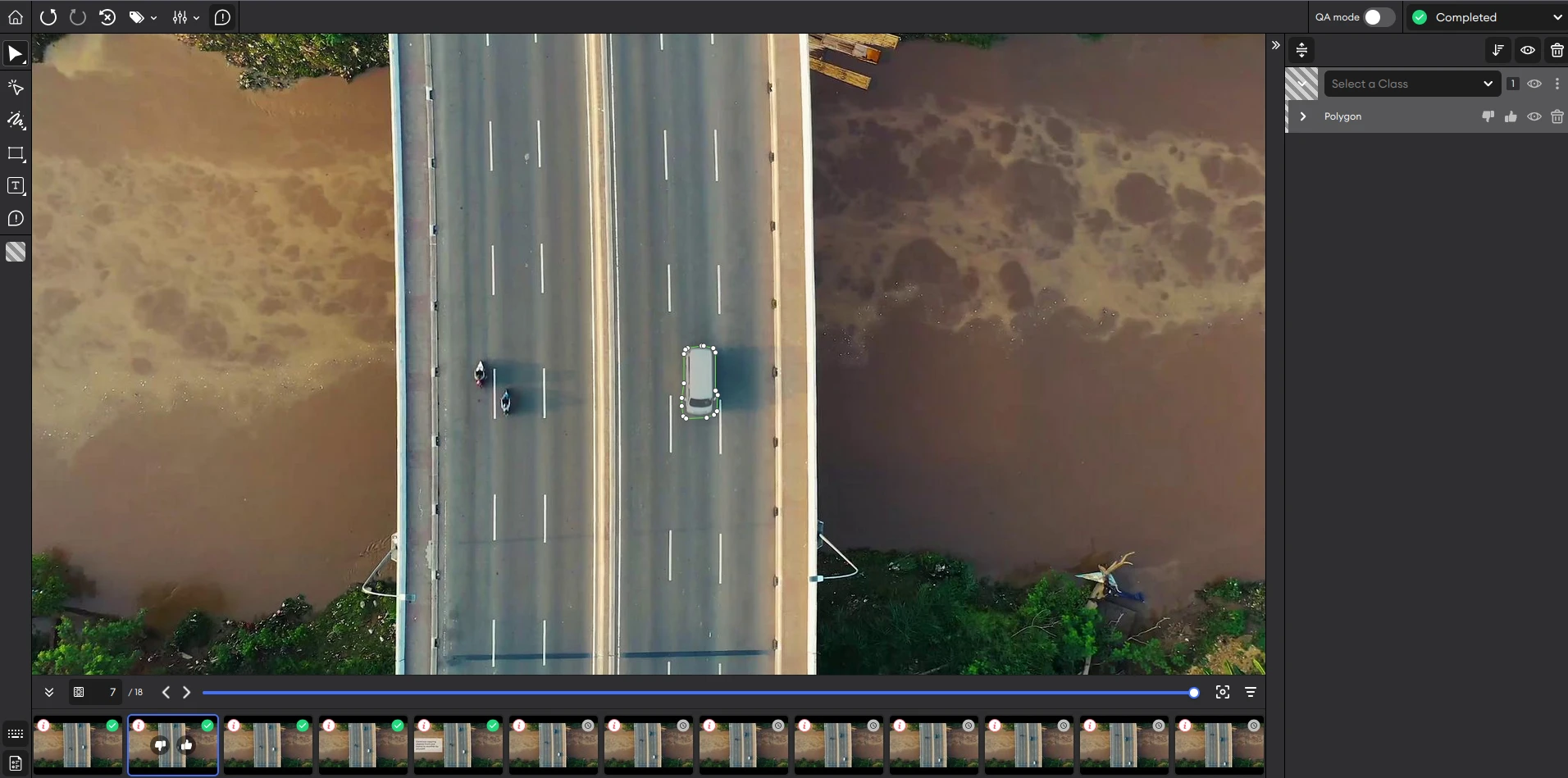
SuperAnnotate
SuperAnnotate is a widely used data annotation platform designed for both manual and automated labeling. It supports a wide range of use cases, from autonomous driving to drone imagery and medical data, providing a comprehensive annotation toolset. Superannotate also has a free Desktop application with a subset of the features available on the online platform.
Segmentation Tools: SuperAnnotate features polygon, brush, and pen tools, giving users the flexibility to create both rough and accurate segmentations. The platform’s advanced editing tools make it easier to refine and adjust segmentations with high precision.
Instance Segmentation Capabilities: SuperAnnotate supports instance segmentation, making it possible to label and differentiate between multiple instances of the same object. This is especially useful in fields like agriculture or aerial imagery, where multiple instances of similar objects need to be tracked.
Automatic Segmentation Features: SuperAnnotate provides several tools for automatic segmentation, including AI-powered features that speed up the annotation process.
Quality Assessment and Assurance Features: For quality control, SuperAnnotate includes features like multi-step QA pipelines, where annotations go through multiple stages of review. Additionally, it offers consensus scoring, where multiple annotators work on the same dataset, and their work is compared to ensure consistency and accuracy.
Supervisely
Supervisely is a robust platform for image annotation, machine learning model training, and data management. It’s widely used across industries, from retail to medical imaging, thanks to its user-friendly interface and support for a wide range of annotation tasks. Supervisely's USP is a wide array of plugins that can be attached to your supervisely workspace to extend the functionality. For many tasks, however, this extensively can sometimes be a bit overwhelming and unnecessary.
Segmentation Tools: Supervisely provides an array of segmentation tools, including the brush, polygon, and pen tools. The intelligent brush tool stands out, as it can adapt its size based on object features, making it easier to annotate complex shapes.
Instance Segmentation Capabilities: Supervisely handles instance segmentation smoothly, allowing for the annotation of multiple objects in a single image. It’s equipped with features like class management, where users can efficiently manage and organize different object classes.
Automatic Segmentation Features: Supervisely offers smart tools for automatic segmentation, which use AI algorithms to predict object boundaries and segment images with minimal human input. The auto-annotation feature enables users to apply AI models to quickly annotate large datasets with high accuracy.
Quality Assessment and Assurance Features: Supervisely’s QA features include error detection tools that flag annotations that fall outside predefined guidelines. The platform also supports collaborative review workflows, where multiple users can review annotations, ensuring that datasets are accurate and reliable before being used to train models.
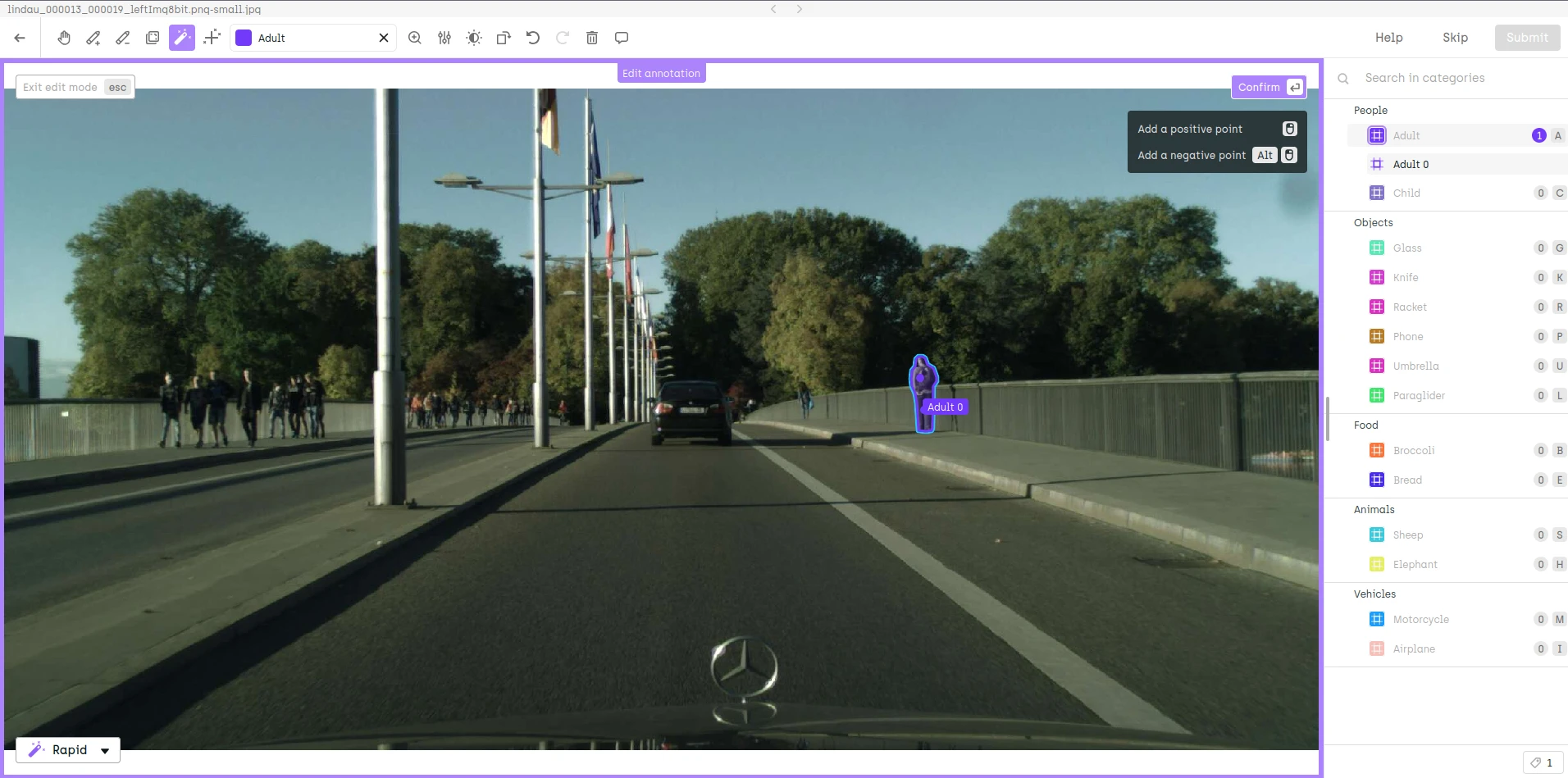
Kili Technology
Kili Technology is a robust data annotation platform that offers solutions for both manual and automated labeling. It’s especially noted for its collaboration features, allowing teams to work together on large annotation projects efficiently.
Segmentation Tools: Kili Technology provides users with powerful segmentation tools like the polygon, brush, and pen tools. These tools are designed for ease of use, making it simple to label even the most complex shapes and objects in images.
Instance Segmentation Capabilities: Kili Technology supports instance segmentation, providing advanced capabilities for labeling multiple objects of the same class within an image. The tool is built to handle detailed labeling tasks, making it suitable for a wide variety of industries.
Automatic Segmentation Features: Kili’s platform is integrated with AI-driven auto-segmentation tools that help automate the process of image segmentation. The AI model integration feature allows users to train and deploy models directly within the platform to predict object boundaries and automate the segmentation process.
Quality Assessment and Assurance Features: Kili Technology places a strong emphasis on quality control. It includes features like automated QA checks, which review annotations for consistency and accuracy. Additionally, collaborative review workflows allow teams to review and validate annotations in real-time, ensuring high-quality output.
Encord
Encord is an emerging player in the image segmentation and labeling industry, offering a scalable and AI-assisted platform designed for industries such as healthcare, robotics, and autonomous systems. It focuses on creating scalable annotation workflows to handle complex datasets.
Segmentation Tools: Encord offers a diverse set of segmentation tools, including the polygon, pen, and brush tools, which can be used to create precise boundaries around objects. The platform’s snapping tools allow for efficient edge detection, reducing the need for manual refinement.
Instance Segmentation Capabilities: Encord handles instance segmentation by enabling users to segment and label multiple instances of objects within an image. Its hierarchical labeling allows for the organization and segmentation of nested objects, which is useful in complex datasets.
Automatic Segmentation Features: Encord integrates AI-powered auto-segmentation capabilities, which automate repetitive tasks like drawing boundaries around objects. It also features pre-trained models that can be applied to new datasets to speed up the segmentation process.
Quality Assessment and Assurance Features: For quality assurance, Encord offers annotation audits where experts can review and flag annotations that need improvement. Additionally, the platform provides performance metrics for annotators, helping teams ensure that their data meets the necessary standards.