What is Data Centric AI?
While algorithms are usually the focus of research in AI, in the real world high quality data matters much more.
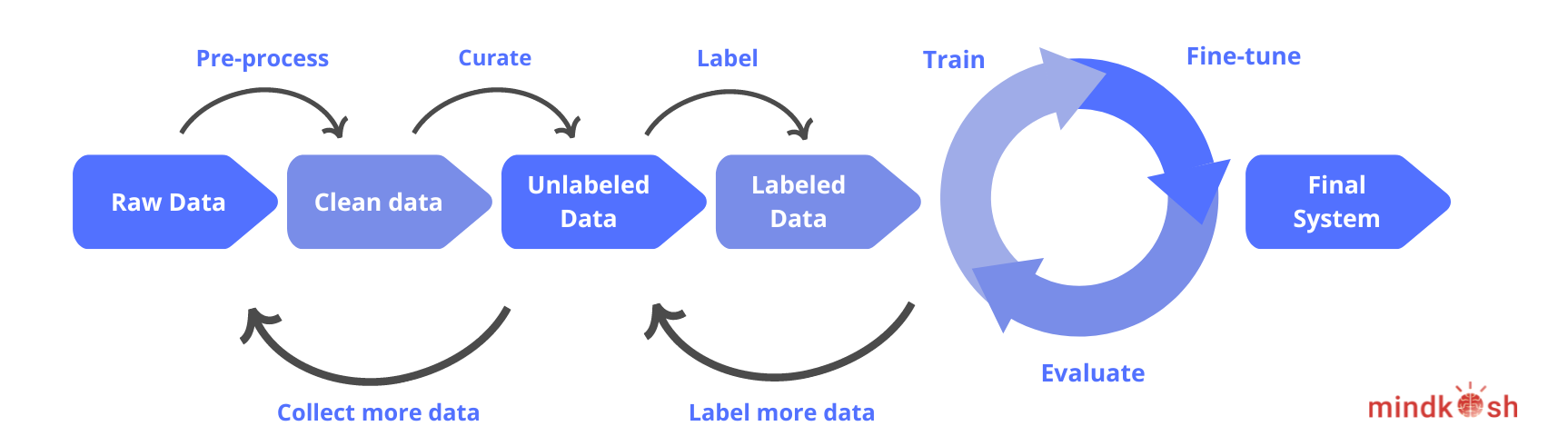
What is Data Centric AI?
The Data Centric AI approach to building AI systems focuses on data instead of code. Traditionally, AI system development involves a Model cycle - continuous testing and adaption of models. The Data centric AI approach adds a Data cycle to the process - continuous improvement of Data Quality.
Why Data Centric AI?
Close to 90% of AI systems organizations work on, are never brought to light. To a large extent, this can be attributed to the fact that real world data can differ significantly from the data in the lab.
Current Machine learning architectures are highly evolved for identifying photographs, recognizing speech, generating text etc. Tinkering with their architecture is perhaps not the best way to improve them anymore.
Data Centric AI can be the answer here.
While Machine learning models have grown more complex, they have also grown more opaque, and require much higher volumes of training data. Data-centric AI promises to unlock higher levels of model accuracy than is possible using plain old model-centric approaches.
Data Centric vs Model Centric approaches
In the traditional model centric approach, ML engineers and Data Scientists largely consider the training datasets from which their model is learning as a static set of ground-truth labels, and the Machine Learning model is made to fit that labeled training data. The training data is essentially considered outside the ML development process.
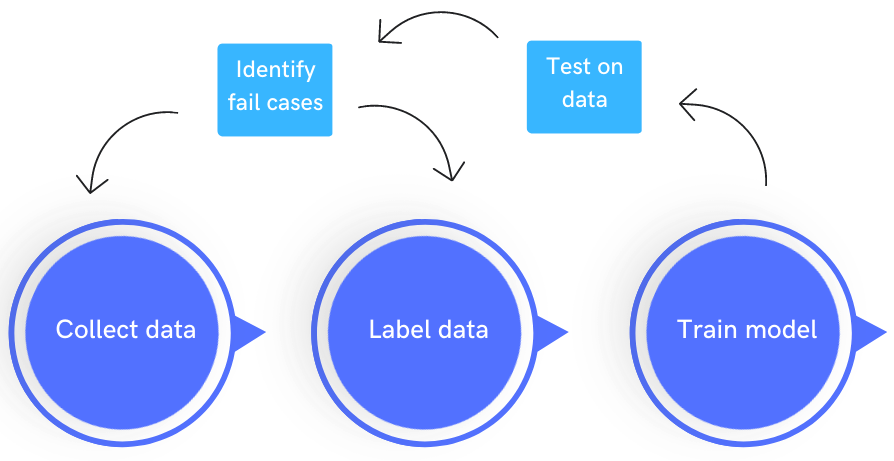
The newer Data Centric approach, is not just a technological shift, but also a cultural shift in the Machine Learning community. In this approach you focus on pre-processing, curating, labeling, augmenting, and managing the data efficiently, with the ML model itself relatively fixed.
It is also important to stress that when developing a ML system, you don't have to choose between a data-centric or a model-centric approache. Building successful AI systems requires both well-conceived models and high quality data.
How does a Data Centric approach benefit me?
More and more of the world’s largest organizations are adopting data-centric AI and seeing considerable benefits. Some of them are:
- Build with less data: Most state-of-the-art Machine Learning models are built to work witg large volumes of data Such volumes are not always available. Specially in industries like manufacturing, where AI is still in the process of making a significant dent. In such cases, a Data centric approach can be helpful as it forces you to focus on the quality of the labeled data.
- Higher quality AI systems: With higher quality labeled datasets, AI systems can achieve higher levels of accuracy than are possible through model centric approaches alone.
- Quicker development: With high quality data, development of AI systems can be done quicker as you can reach a desired level of accuracy relatively quickly.
- Lower costs: With systematic development of labeled datasets, organizations spend less time on the development and need fewer labeled examples. Resulting in lower costs overall.
Our commitment towards Data Centric AI
To learn more about what features we have panned for the future, see our Trello feature board. If you find something you would love to see us build, feel free to like the features and comment on them to let us know! We are listening.