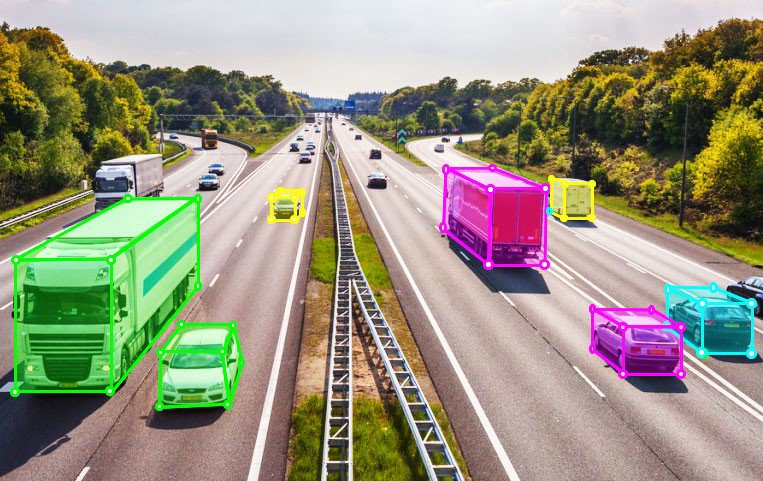
Data Annotation: A Brief Introduction
The categorising and labeling of data for AI applications is known as data annotation. For a specific use case, training data must be correctly classified and annotated. Companies may establish and improve AI solutions by using high-quality, human-powered data annotation.
To know more about Data Annotation, you can check our blog on Data Annotation and Its Use Cases
Let us now have a look at 2D and 3D Data Annotations and understand them in more detail.
2D Data Annotation And Its Use Cases
For 2D Image annotation, a rectangle or square is drawn around the object of interest with the goal of identifying it. To make the object recognizable for machine learning training, co-ordinates of the corners of the rectangle/square are collected.
Depending on the needs of the annotator, colored or un-shaded boxes are generated while drawing the 2D picture annotation, to make the boxes clearly visible. 2D bounding box annotation is fairly simple to create, and there are a variety of tools or software available to make it easier for annotators.
Some Common Use Cases of 2D Annotation
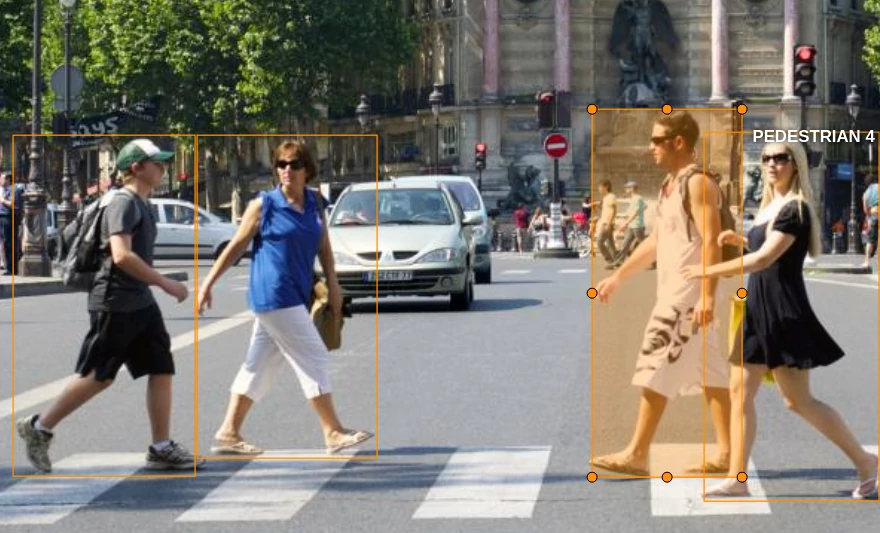
2D bounding box annotation is widely used in autonomous driving to train autonomous vehicles to detect various objects on the streets such as lanes, traffic, potholes, signals, and other objects. One of the most basic ways for determining attributes for computer vision-based models is bounding box annotation. The bounding box annotated photos are used to educate autonomous vehicles to detect various objects on the roadway, such as traffic, lights, lanes, and potholes, among other things. In a real-world setting, it also allows self-driving vehicles to detect and understand their environment.
2D bounding box annotation is also utilised in AI systems designed for other industries like retail, healthcare and agriculture to recognise items
While the task appears straightforward at first, maintaining consistency necessitates dedicated efforts.
An Introduction To 3D Data Annotation
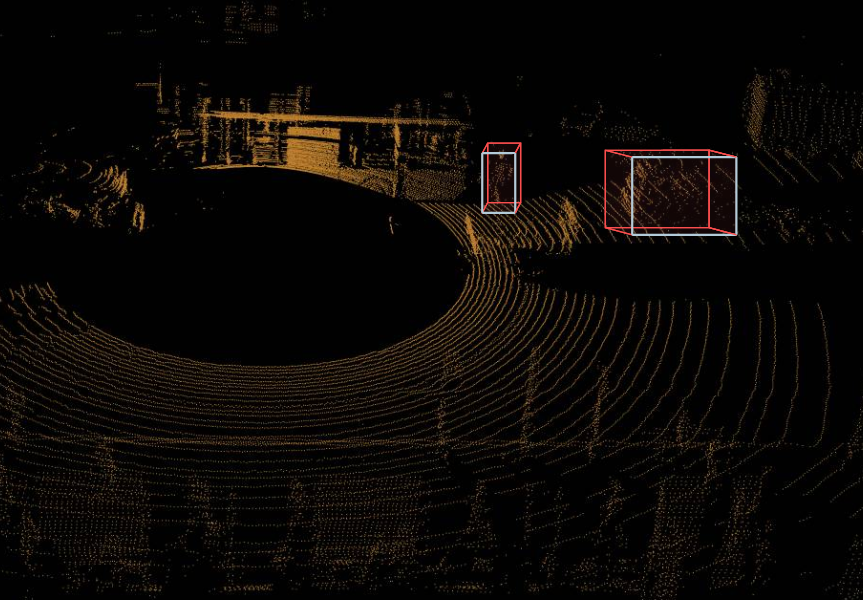
3D annotation is generally performed on three-dimensional data such as point clouds. It allows for depth, distance, and volume to be taken into account. This is most often done with cuboids instead of squares/rectangles, but it can also be done by using voxels (3D pixels) for semantic segmentation of the pointclouds.
Some common Use Cases of 3D Data Annotation Include:
- Medical: Interpreting CT and MRI scans in 3D.
- Geospatial: Recognising 3D structures using Synthetic-Aperture Radar imagery.
- Autonomous vehicles: Vehicle tracking based on LiDAR point cloud data.
- Manufacturing: Detecting irregularities through 3D images of products.
- Agriculture: Detecting ground topology.
- Retail: Detecting gestures and attitudes for VR and AR applications.
Benefits
This method of data annotation considers more dimensions and hence delivers more meaningful data, particularly in conditions that impact 2D imagery (e.g. fog, darkness, occlusion, etc.).