As AI continues to permeate all aspects of our daily lives, high-quality data has become one of the most sought after resources. Data annotation, the process of labeling data to train Machine Learning models, is the most important step in any data pipeline. However, it is often outsourced to human annotators, raising significant ethical concerns regarding their rights, working conditions, and mental well-being. We inspect all these aspects in this article.
Hiqh quality annotated data is key to AI
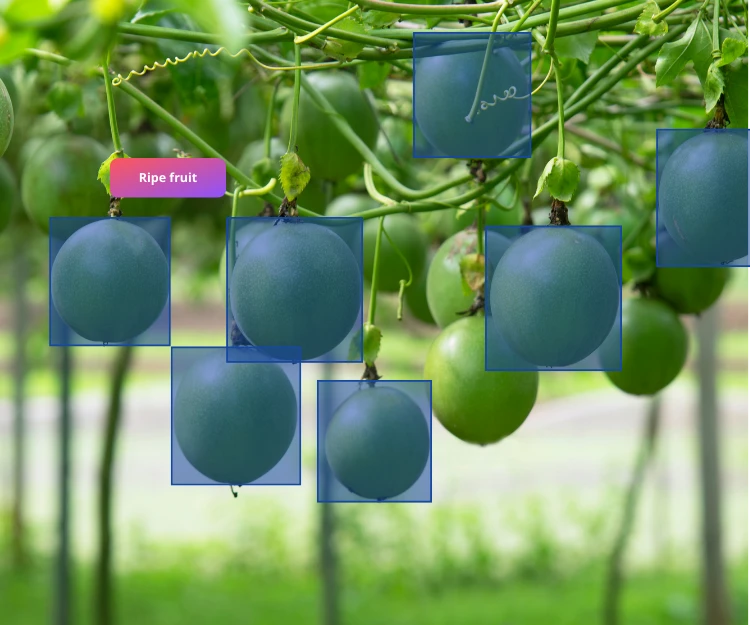
Data annotation directly impacts how well an ML model performs in the real world. In many ways, the quality of the annotated data outlines what an AI system can and cannot learn. For example, in tasks like object detection or language translation, if the annotations are inconsistent or incorrect, the model’s training will be skewed, leading to unreliable predictions.
In some cases, even subtle differences in how data is annotated can significantly shift model behavior. For example, when developing ML systems for Autonomous vehicles, mislabeled pedestrians or vehicles in the training set can lead to real-life safety risks on the road. Ensuring high quality annotations is absolutely key in such scenarios.
High quality data annotation isn’t just about getting the labels right—it’s about getting them consistently right over a massive dataset, ensuring that the model develops a robust understanding that applies across diverse scenarios.
Rise of large scale Data Annotation efforts
With the recent boom in AI and its applications, Machine Learning has quickly moved from niche applications to widespread adoption. As a result, the demand for large-scale annotated datasets has also exploded. Whether it's for Generative AI, Computer vision, or Autonomous systems, the amount of data needed to train reliable models keeps growing.
Large-scale annotation efforts are driven by the need for diversity in datasets. For instance, a ML model trained solely on data from a single region or demographic will have a limited understanding of the world. To tackle this, companies are now looking to annotate vast amounts of data that reflect various cultures, geographies, and contexts. These efforts are both labor-intensive and critical for developing AI systems that work in the wild, not just in controlled environments.
In response, we’ve seen a significant expansion in data annotation workflows—outsourcing to a global workforce, crowdsourcing platforms, and the development of semi-automated tools designed to scale up the annotation process. Some ML teams have turned to hybrid systems, merging human annotators with automated labeling workflows to speed up the process while maintaining Quality Control.
Poor working conditions for data annotators
The human side of all this data is often ignored. For many data annotators, the work environment can be grueling. Long hours of sitting in front of a screen, repeatedly ranking LLM responses or classifying images, leads to physical fatigue and mental burnout. The tasks themselves are often highly repetitive—whether it’s marking the boundaries of objects in thousands of images or transcribing endless hours of speech data, the work can get very monotonous. Over time, this kind of routine work can wear down even the most patient workers.
But what makes the situation untenable is that many annotators work under tight deadlines with little to no control over their schedules. Annotation is often outsourced to countries where labor is cheap, and as a result, annotators might work in sub-optimal conditions, with inadequate breaks, poor equipment, and minimal job security. In extreme cases, vendors push for productivity over well-being, setting quotas that can lead to overworked and underpaid annotators. Real-life reports from countries like the Philippines and India highlight workers earning low wages for incredibly demanding, tedious tasks, sometimes without the option to voice concerns due to contractual limitations.
Psychological toll from sensitive or explicit content
Another serious issue is the psychological impact of dealing with disturbing or explicit content. Many annotators are required to review and label graphic images, violent scenes, or sexually explicit material, especially for content moderation tasks. Prolonged exposure to such content can lead to severe psychological effects, including stress, anxiety, and post-traumatic stress disorder (PTSD).
Take, for example, the case of Facebook's content moderators, who sued the company over claims of PTSD and other trauma-related conditions after having to review violent and graphic posts for hours on end. These moderators had to sift through videos of assaults, suicides, and other graphic material as part of their job, with little to no mental health support from their employers. This situation is not unique to social media platforms—data annotators working on AI training sets for law enforcement, healthcare, or autonomous systems often encounter similarly distressing material. For many annotators, data annotation can be the source of their primary income, so they feel they don't really have the choice to pick and choose project.s
Without proper psychological support or the option to refuse certain types of tasks, annotators can become desensitized or suffer from emotional exhaustion. The constant exposure to graphic content doesn’t just affect job performance—it can have long-term effects on mental well-being.
Improving working condition for data labelers
One of the most straightforward ways to improve the lives of data annotators is ensuring fair compensation. Many annotators, especially those in outsourced roles, are underpaid for the amount of labor and focus their jobs require. Companies need to move beyond the "low-cost" labor model and pay wages that reflect the mental effort and time involved. This means not just matching local minimum wages but offering pay that incentivizes quality work and acknowledges the expertise required.
Equally important is setting reasonable work hours. Annotation tasks are mentally taxing, especially when they’re repetitive or require intense focus. Stretching these tasks over long shifts not only hurts annotator productivity but also leads to burnout. Companies should prioritize regular breaks and enforce manageable working hours to maintain a sustainable workflow. A good practice would be to align annotation tasks with productivity windows where workers can perform at their best without exhaustion.
Beyond financial and scheduling considerations, mental health support is also very important. Annotation jobs often involve high-pressure environments, and burnout is common when mental health is neglected. Offering access to counseling, mental health days, and stress management resources can make a significant difference. Some companies are now starting to offer these kinds of programs, recognizing that mental well-being directly impacts the quality of the work.
Protection against psychological impact
For annotators tasked with labeling sensitive or explicit content, it is imperative to protect against the psychological impact of the work. Companies should first consider filtering tasks so that sensitive content is handled by those who are better equipped to manage the mental load, either through training or voluntary participation. No one should be forced to review disturbing material without consent or adequate preparation.
Wherever possible, automatic filtering should be used to make sure only content that absolutely needs to be reviewed by humans is exposed to annotators. For example, both Meta and Youtube use few-shot detection to filter toxic content and assign a degree of certainty to the content. Only content that is either low in confidence is sent for human review.
When annotators do need to interact with harmful content, there must be proper safeguards in place. Content moderation teams, for example, can be rotated more frequently than normal, so that individuals aren’t exposed to harmful material for extended periods of time. In addition, offering trauma-informed mental health support, such as regular check-ins with therapists or creating peer support groups, can mitigate long-term effects.
A good real-world example is the content moderation lawsuit against Facebook mentioned earlier. Facebook ultimately had to agree to improve mental health benefits and change working conditions after it became clear the existing protections were insufficient. This should set a standard across industries that rely on human reviewers for sensitive data.
Escaping the race to the bottom
When choosing Data annotation vendors, the comparison points are often cost and speed. To compete on those terms, some vendors take shortcuts that can achieve lower prices, but through unethical treatment of the annotators. This leads to a "race to the bottom" where the only aspects left to compete on are speed and cost, at the expense of both - quality of data as well as the well-being of data annotators.
From the end-user's perspective, if an ML team wants to choose a vendor who isn’t the lowest bid, they often need to justify their choice to another team, such procurement. In such a case, a scorecard that can objectively measure a vendor against a set of standard criteria can make it easier to explain why spending additional money is appropriate, in pursuit of essential high-quality data. Here is an interactive tool to assign a numerical score to annotation vendors, based on the most important aspects of a data annotation project.
The tool has been adopted from the paper "Towards a Shared Rubric for Dataset Annotation" by Andrew Marc Greene.
Legislation and Industry standards
While millions of people around the world engage in data annotation, the industry remains largely unregulated, leading to potential exploitation. Legislation and industry standards can play an important role in safeguarding the rights and well-being of data annotators. Governments and industry bodies must come together to establish regulations that enforce fair labor practices, protect mental health, and ensure ethical data handling.
Initiatives like the AI Ethics Guidelines by the European Commission and efforts by organizations like the Partnership on AI to promote fair labor practices are steps in the right direction. However, continuous efforts are needed to adapt these regulations to the quickly evolving landscape of AI and data annotation.
Data annotators are the unsung heroes of the AI industry. Their work ensures that Machine Learning models function correctly, but their contributions are often overlooked and undervalued. We need to ensure that annotators' rights are protected, including fair wages, reasonable working hours, and job security. The future of AI depends not only on technological advancements but also on our commitment to ethical practices and human rights. After all AI, and technology in general, is supposed to serve human endeavors, not the other way around.