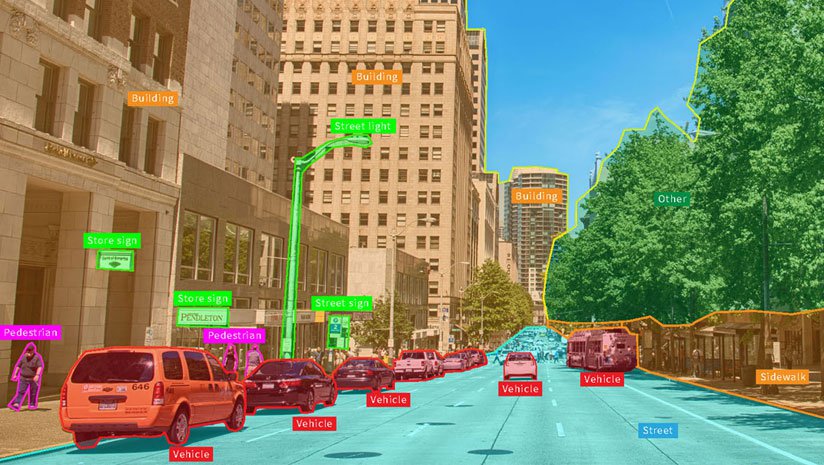
Managing and streamlining a data annotation process is difficult. Businesses face a number of internal and external challenges that make the work of annotating data inefficient and ineffective. Is outsourcing a solution to these challenges? First let's understand what problems an organization is likely to face when handling data labeling projects in-house.
Managing a large labeling workforce
Most Machine Learning and Artificial Intelligence models need a huge amount of labeled data to learn. Businesses need to employ a sizable staff to manually annotate the datasets in order to provide the vast amount of annotated data needed to train these AI/ML models. Additionally, analyzing big data and annotating them with the best quality is crucial to achieving a high level of accuracy. But managing such a sizeable workforce is a big challenge for the management. Organizational problems can affect a company's effectiveness, productivity, and quality.
In addition, for most organizations, this management is outside the scope of their core activities. So while it is possible to hire and maintain a large workforce if you consistently need large annotated datasets, it is not advised, as it distracts your team from performing their most important day-to-day activities.
Lack of access to proper data labeling tools and software
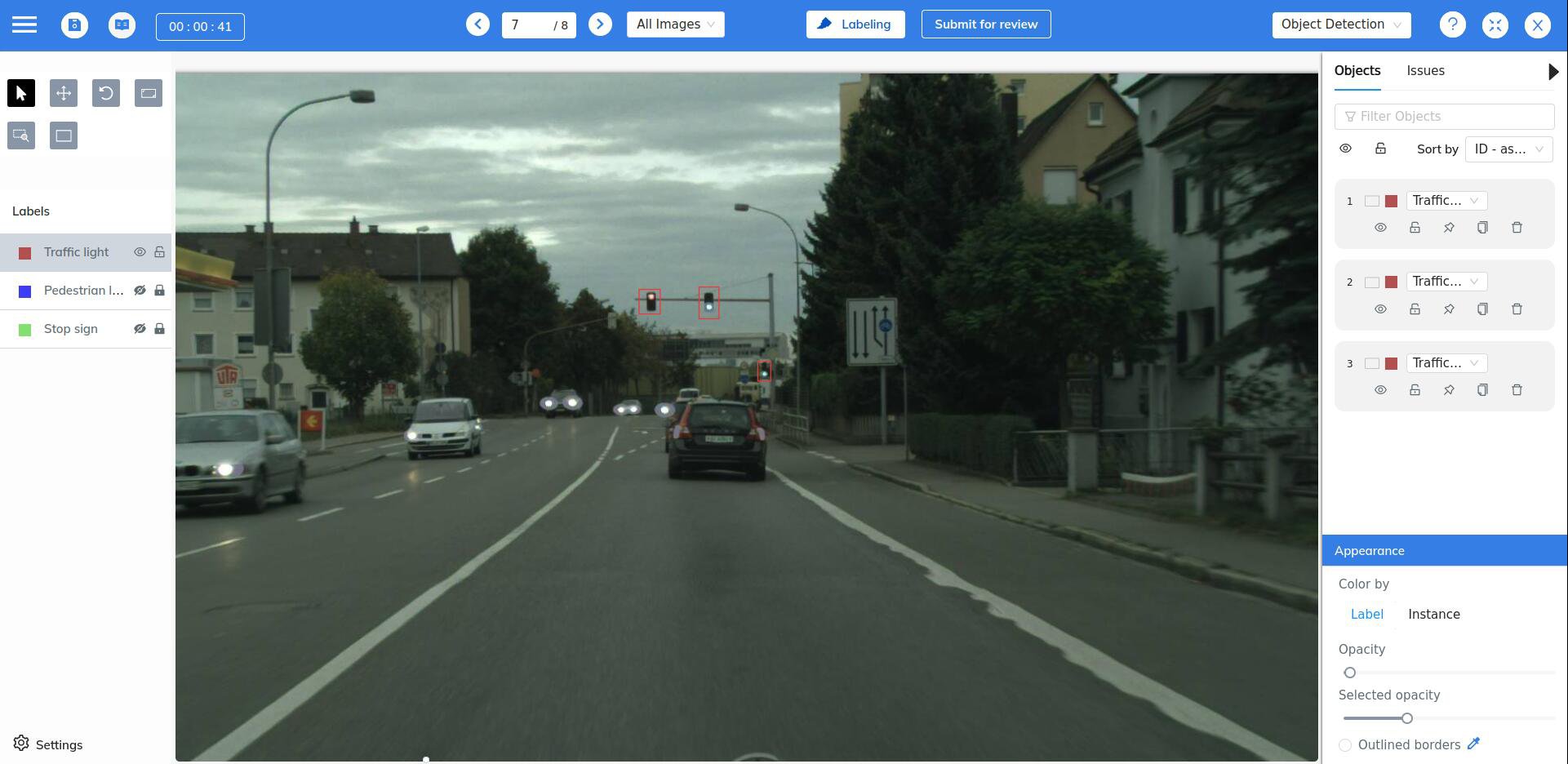
Having a large and skilled workforce does not guarantee the production of high-quality labeled datasets. To carry out an accurate data labeling process, the right equipment and technology are needed. Different technologies and methods are employed to label data for deep learning depending on the type of data. But organizations frequently fall short in creating the infrastructure necessary for the best possible labeled datasets.
Developing the required tools and software in-house is expensive. And so is maintaining them. In addition, organizations dedicated to building tools for Data labeling have far more experience and expertise in data labeling, and understand the difficulties faced in producing large volumes of labeled datasets. This allows them to build tools that not only help in efficiently label data, but also to make sure the quality of the labels is very high.
In some cases, deploying freely available tools might satisfy the needs. But for most data types other than images, the available tools either simply don't exist, or are too lightweight to be useful for anything other than experiments and small projects. Even for image based datasets, managing a large workforce and large volumes of data can be cumbersom with the most popular publicly available tools.
Difficulty in producing consistently high quality data labels
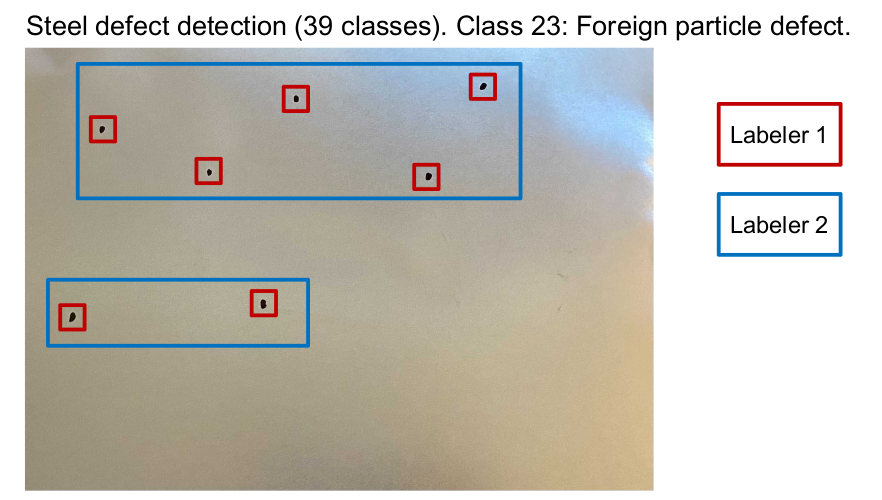
AI/ML models that actually work in the real world necessitate the highest quality labeled datasets. There is very little room for errors. Small mistakes can have a major impact on a system's output. There are a few common causes for low quality labeled datasets:
- Careless errors - Humans make mistakes. No matter how careful we are. A good labeling tool will try to reduce the possibility of this happening by catching common mistakes and making it easy to audit and validate labels.
- Inconsistent labeling among labelers - When there is a large workforce involved, it is common for different people to interpret the same labeling instruction differently. Thus they end up labeling similar situations differently.
- Incorrect understanding of labeling instructions - Another problem associated with a large workforce is an improper understanding of labeling instructions. The biggest issue here is that errors are only caught during validation by which time a lot of time and effort is already wasted.
Furthermore, obtaining consistently high-quality data is a big challenge in itself. It is essential for organisations to keep a steady flow of high-quality, labeled datasets for machine learning training and accurate AI prediction. Obtaining such datasets can be a daunting task for organizations to do in-house.
Can outsourcing data labeling help solve these problems?
Outsourcing your data labeling projects can be helpful as you struggle to meet data labeling standards and project deadlines. Let’s see how.
Maintain high quality of labels throughout the dataset
Extremely accurate and high-quality training data sets are essential to every ML model's success. The success of the project may depend on the accuracy of the data labeling. The key advantage of outsourcing data labeling solutions is that qualified and seasoned people are brought on board by the outsourced partner. As they are used to processing large volumes of dara, they work more quickly and precisely than internal teams. As a result, service providers guarantee the highest level of precision while retaining efficiency and staying on schedule for the project.
A good oursourcing partner also has access to specialised labeling tools which allows them to catch errors early or to avoid them completely. At Mindkosh we have been hard at work developing our data labeling tool that catches mistakes early, facilitates smooth communication among all stakeholders, and utilises semi-automatic labeling using AI to produce consistently high quality labels.
Scale up and down quickly
Millions of annotated data points are often needed in order to successfully train a deep learning model. Businesses can fall short of finishing massive labeling projects due to a shortage of staff because the demand fluctuates, sometimes being extremely low and sometimes being very high.
Such problems can easily be solved by outsourcing partners. They can offer competent and skilled annotators on demand to handle a sizable number of data labeling assignments. They can scale up as well as down without sacrificing quality and have the bandwidth to accommodate various labeling requirements.
Meet project deadlines
Total reliance on an internal workforce for data labeling could push back the deadline since they are only required to work during office hours. Additionally, managing, onboarding, and training annotators might take time. Because of this, it lacks urgency and offers a slower rate of project completion.
A difference of weeks or even months can be made by outsourcing data labeling to a professional service provider with a highly skilled and motivated workforce. As a result, you never fall behind schedule.
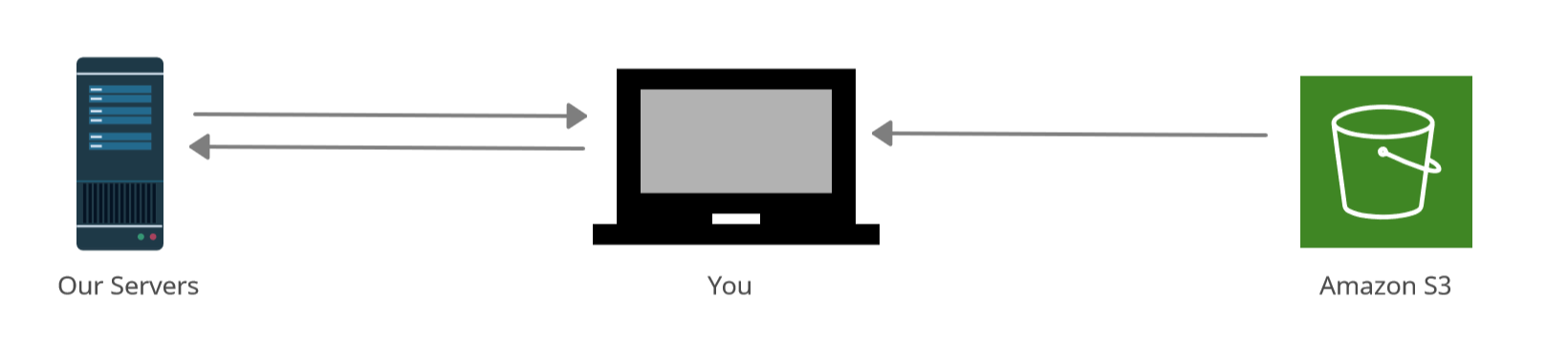
Is my data secure when oursourcing data labeling?
While it is clear outsourcing data labeling can be very benefitial, a common refrain from companies when thinking about outsourcing is - Is my data secure when outsourcing for data labeling? Mindkosh provides several solutions to solve this problem.
From the ground up Mindkosh is built to keep your data extremely secure. All workplaces have security cameras and strict policies around carying cellphones and storage devices. All data is kept securely in AWS S3 buckets with regular backups. You also have the option to stream your data directly from your own S3 accounts. In this case your data never passes through our servers and is streamed directly to the labeler's browser.
If you are required to keep your data within your premises because of regulatory hurdles, we also support deployment of our software tools on your infrastructure so that your data never leaves your organization. Get in touch with us for more details.
And remember - "Garbage-in garbage-out" !