Ever wondered how suspicious activities are detected by security cameras, or how your favorite cricket teams analyze game footage? The answer is a Machine Learning technique called Computer Vision, powered by Video annotation, which helps Machine Learning systems learn whatever it is they need to do. But what exactly is video annotation, and why is it important?
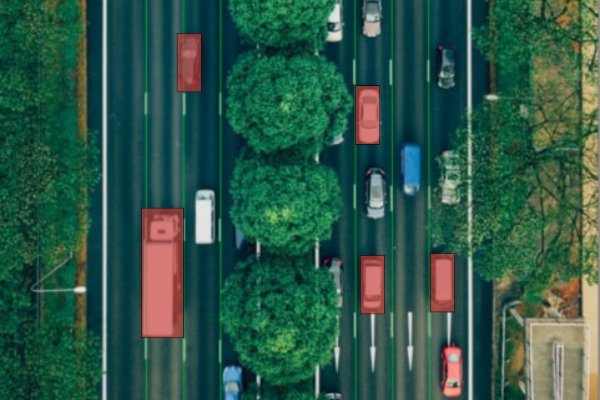
What is Video Annotation?
Video annotation or video labeling, involves adding annotations to videos to help AI-powered systems in identifying objects precisely. This process is important for training models to recognize all sorts of things in videos like cars, plants etc. With the increasing demand of computer vision in various tasks, the significance of video annotation cannot be overstated.
To accelerate the annotation process and manage large datasets efficiently, video annotation tools are used. These tools provide high accuracy and consistency in labeling, enabling annotators to steer through complex video data effectively.
Types of Video Annotation
1. Bounding Boxes
Bounding boxes are one of the simplest and most widely used annotation techniques. Annotators draw rectangular boxes around objects in a video frame. This is perfect for object detection tasks where the goal is to identify and track the presence of specific items, such as cars, people or animals. Bounding boxes provide a clear and concrete way to define an object's location.
2. Polygons
For more complex shapes that don’t fit neatly into a rectangle, polygon annotation is the answer. This technique involves drawing multi-sided shapes around the object, closely fitting its contours. Polygons offer a higher level of precision, which is particularly useful in fields like agriculture, where objects such as leaves or fruits need to be accurately outlined. This method captures the exact shape and size of an object, providing more detailed data for analysis.
3. Polylines
Polylines are used to annotate linear structures. Annotators draw lines to represent objects like roads, pipelines, or boundaries. This technique is essential for applications in mapping and navigation, where understanding the layout of linear features is critical. For example in self-driving cars, polylines help the vehicle's AI understand the road's edges and lanes ensuring safety navigation.
4. Keypoints
Keypoint annotation involves marking specific points of interest on an object, such as the eyes, nose or mouth. AI models can analyze human movements and expressions by identifying and tracking the above-mentioned key points, which is valuable in sports analytics, virtual reality, and animation.
5. 3D Annotation
3D annotation adds a new dimension to the labeling process, allowing objects to be identified in three dimensions. For models that require spatial awareness such as automatic vehicles and robotics 3D annotation is the solution. With 3D annotation, systems can understand the depth and distance between objects, which is essential for navigating and interacting with the real world safely and efficiently.
6. Semantic Segmentation
Semantic segmentation takes annotation to a pixel level, dividing the entire frame into segments and labeling each pixel according to the object class it belongs to. So, it provides a detailed understanding of the scene, making it invaluable for applications requiring precise object and scene recognition. It is widely used in medical imaging thereby helping to differentiate between various tissues and organs, aiding in accurate diagnosis as well as planning of treatment.
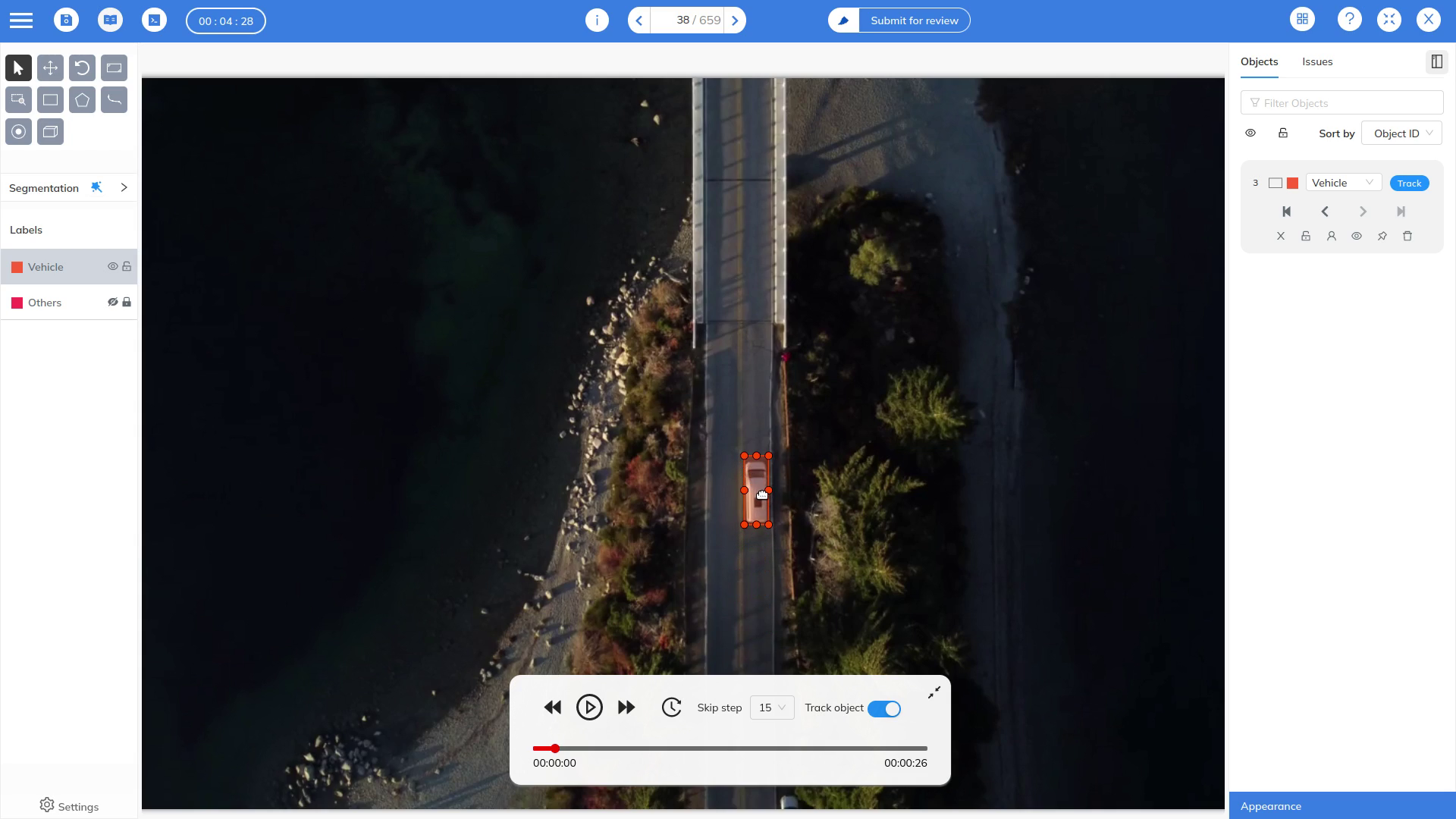
A Step-by-Step Guide to Annotating Videos for Computer Vision Model Training
1) Define the Objective
Before you start annotating, clearly define what you want your computer vision model to learn. Are you focusing on object detection, tracking, or action recognition? Knowing your end goal will guide your annotation process and help you choose the right techniques and tools.
2) Choose the Right Video Annotation Tool
When selecting a video annotation tool, consider the type of dataset and techniques you'll use. Look for these features to facilitate annotation:
- Advanced Video Handling: Ensure compatibility with diverse formats.
- User-Friendly Interface: Opt for an intuitive tool.
- Dynamic Classification: Choose tools supporting event-based labeling.
- Automated Tracking: Look for features like object tracking.
- Team and Project Management: Consider tools with collaborative features.
At Mindkosh, we offer a robust inbuilt video annotation tool designed to handle various annotation tasks efficiently. Our tool supports multiple annotation types and provides an intuitive interface for seamless workflow management. You can learn more about our platform here.
3) Review and Refine Annotations
Regular review of annotations is essential to uphold dataset accuracy, particularly with large datasets prone to errors or omissions. Annotation tools provide operation dashboards for progress monitoring and issue identification, while automating pipelines ensures continuous, scalable data delivery. By regularly reviewing annotations, organisations can maintain dataset integrity and ensure machine learning models trained on them perform optimally.
This process safeguards against inaccuracies that could compromise model performance, thereby enhancing the reliability of annotated datasets. Additionally, automation streamlines the review process, allowing for efficient management of expanding datasets without sacrificing quality.
Top 5 Use Cases of Video Annotation
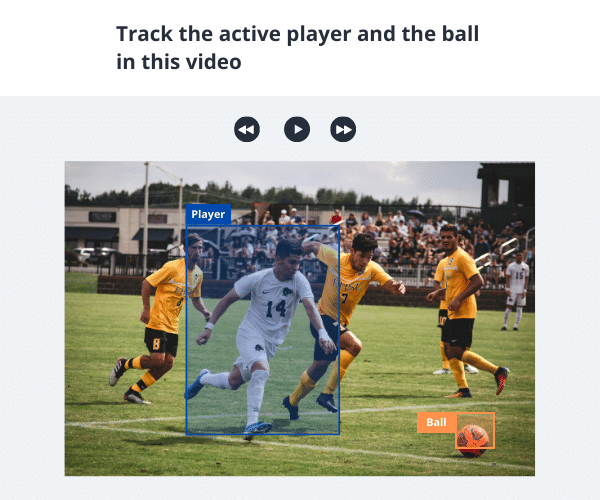
Autonomous Vehicles
Video annotation helps in the development of machine learning models for self-driving vehicles, enabling them to identify objects on the road and nearby vehicles. Moreover, it also contributes to building collision avoidance systems, enhancing vehicle safety.
Surveillance Systems
In surveillance systems, annotated videos are instrumental in identifying and tracking suspicious activities or individuals. Video annotation enhances situational awareness and aids in forensic analysis.
Medical Imaging
In Medical Imaging, video annotation helps medical professionals in understanding imaging data such as MRI scans or X-rays for accurate diagnosis and treatment planning. Apart from this,it also enables the identification and classification of abnormalities and anatomical structures.
Sports Analytics
In sports analytics, video annotation enables coaches and analysts to dissect gameplay, track player movements, and identify performance trends. This data-driven approach enhances player training, strategy development, and overall team performance.
Retail and E-Commerce
Video annotation is used in retail and e-commerce to analyze customer behavior, track foot traffic in stores, and optimize store layouts for better customer engagement. It also facilitates product recommendation systems based on customer preferences and interactions.
Enhancing Video Annotation: Key Tips for Success
Accuracy in labeling video datasets is vital for training dependable machine learning models. While choosing the right annotation technique is important, effective video annotation involves more than just that.
So, how can you annotate videos effectively? Here are essential tips to improve your video annotation skills.
Dataset Quality Assurance:
Before annotation begins, ensure dataset cleanliness by removing low-quality and duplicate data. Utilise lossless frame compression in annotation tools to maintain dataset integrity.
Precise Labeling Practices:
Understand the intended use of the dataset in ML model training. Employ appropriate annotation techniques such as bounding boxes for object detection or class labels for classification tasks.
Organized Label Structures:
Implement customized label structures and accurate metadata to prevent misclassification post-annotation. Predefined label structures and corresponding classes for streamlined annotation.
Efficient Annotation Techniques:
Identify keyframes in videos to annotate predictable object movements efficiently. Utilise interpolation techniques to annotate based on keyframes, expediting the annotation process while ensuring accuracy.
User-Friendly Annotation Tools:
Opt for annotation tools with user-friendly interfaces and powerful features. Look for automation capabilities, such as auto-segmentation, to enhance efficiency without compromising precision. Choose tools that align with annotation goals and streamline the annotation process effectively.
Emerging Trends in Video Annotation
Integration of AI and Machine Learning:
The future of video annotation lies in the integration of AI and machine learning. AI-driven algorithms will play a pivotal role in automating annotation processes, reducing manual effort and improving accuracy. Machine learning models will be trained to recognize and annotate objects in videos, enhancing efficiency and scalability.
Advancements in Automated Annotation Tools:
Rapid advancements in automated annotation tools are reshaping the landscape of video annotation. These tools leverage AI and machine learning to automatically detect and label objects in videos, minimising the need for manual intervention.
Features like object tracking, semantic segmentation, and event detection are becoming more sophisticated, enabling precise annotations in diverse video datasets.
Potential Impact on Various Industries:
The integration of AI-driven video annotation technologies is poised to have a transformative impact across various industries. In sectors such as autonomous vehicles, healthcare, surveillance, retail, and entertainment, accurate and efficient video annotation is essential for training machine learning models.
These industries stand to benefit from improved automation, enhanced data quality, and accelerated innovation driven by advanced video annotation techniques.
Video annotation is essential for transforming raw video into actionable data for AI systems. Techniques like bounding boxes, polygons, and 3D annotation enable computer vision models to accurately identify and analyse objects and actions, driving advancements in autonomous vehicles, surveillance, sports analytics, and medical imaging. The rise of AI-driven annotation tools is streamlining this process, reducing manual effort, and enhancing accuracy and scalability.
As industries adopt these advanced methods, video annotation's impact will continue to expand, fostering innovation and improving AI capabilities. By adopting the latest techniques and tools, we can develop smarter, more responsive AI systems, shaping the future of technology and its applications in everyday life.