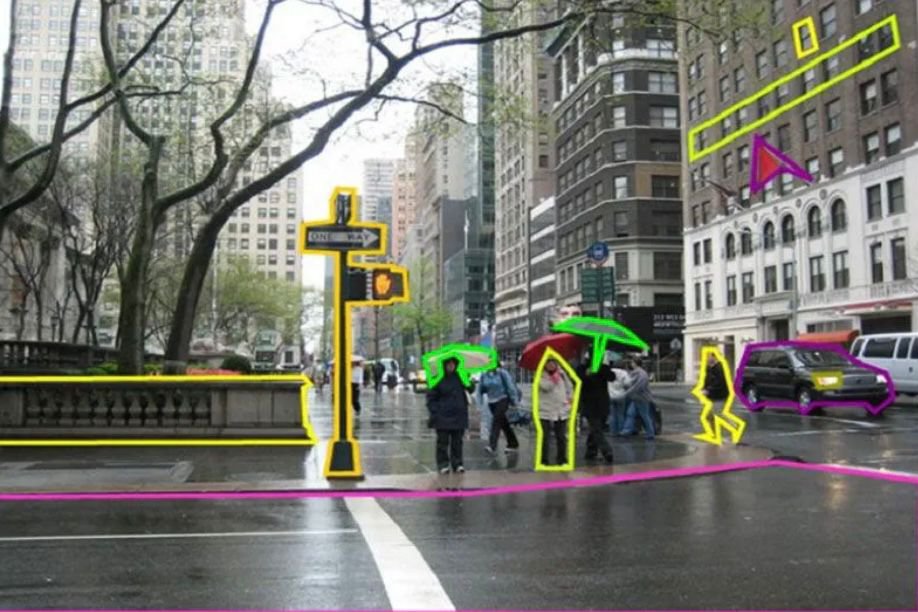
Machine learning has revolutionized almost every industry, from healthcare to autonomous driving. However, the success of Machine Learning models heavily relies on high-quality annotated data. Data annotation, the process of labeling data for training machine learning algorithms, plays a vital role in the development of accurate and reliable models. To streamline this process, data annotation tools have emerged, offering powerful capabilities to annotate data efficiently and effectively.
On the other hand MLOps aims to streamline the entire lifecycle of machine learning models, bridging the gap between developing a model and putting it to use in the real world. We explore both in the sections below.
How Does Data Annotation Work?
Data annotation is the process of labeling datasets to make them understandable to Machine Learning models. It starts with the collection of raw data, which could be anything from text and images to videos or 3D point clouds. This data needs to be carefully labeled to provide context to the model, enabling it to learn patterns and make accurate predictions.
The first step typically involves defining the Annotation guidelines. These are the rules that annotators will follow to label the data consistently. For example, in image annotation, guidelines might specify what objects should be labeled, how to handle overlapping objects, or how to deal with uncertain cases. Clear guidelines are crucial for ensuring that the data is labeled consistently across the dataset.
The next step is the actual annotation process. Depending on the complexity of the task, this might involve a variety of techniques. In simpler cases, it could be as straightforward as tagging objects in an image. For more complex tasks, such as 3D point cloud annotation, annotators might need to define cuboids, segment regions, or even classify individual points. The tools used for annotation often provide specialized features to streamline this process, such as automation tools that assist with repetitive tasks or validation checks that flag potential errors.
Once the data is annotated, it's typically subjected to a Quality Assurance (QA) phase. This step is essential to catch any inconsistencies or errors in the annotations. QA can involve spot-checking samples, running automated validation scripts, or even re-annotating certain portions of the dataset. The goal here is to ensure that the final annotated dataset is as accurate and consistent as possible, as even small errors can significantly impact the performance of the machine learning model.
Finally, the annotated data is formatted and packaged for use in model training. This step might involve converting the annotations into a specific format that the Machine Learning pipeline can process, or splitting the data into training, validation, and test sets.
The Need for Data Annotation
Machine learning models require large amounts of labeled data to learn patterns and make accurate predictions. Annotated data provides the necessary ground truth for training these models. For example, in object detection tasks, images need to be annotated with bounding boxes around objects of interest. In natural language processing, text needs to be annotated with named entities or sentiment labels. The accuracy and quality of annotations directly impact the performance of machine learning models.
Manual annotation can be a time-consuming and labor-intensive process. It requires human annotators to meticulously label each data point based on specific guidelines. Additionally, maintaining consistency and inter-annotator agreement becomes challenging as the annotation complexity increases. This is where data annotation tools come into play, providing features that facilitate and automate the annotation process.
Data Annotation Tools: Features and Capabilities
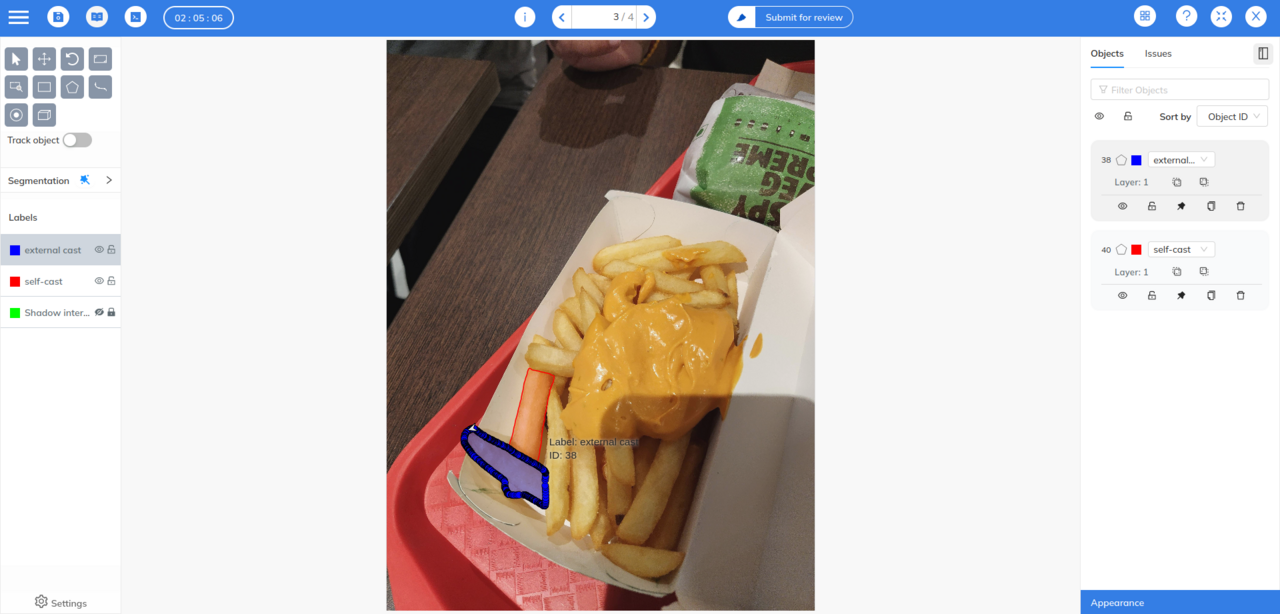
Data annotation tools offer a wide range of features and capabilities to streamline the annotation process. Let's explore some key functionalities commonly found in these tools:
Annotation Interfaces: Data annotation tools provide intuitive user interfaces that allow annotators to label data efficiently. These interfaces often include options for drawing bounding boxes, polygons, or points, depending on the annotation task. They may also support keyboard shortcuts and customization to enhance the annotator's productivity.
Collaboration and Workflow Management: Many annotation tools support collaboration features, enabling multiple annotators to work simultaneously on a project. These tools provide mechanisms to assign annotation tasks, track progress, and resolve conflicts. Workflow management features help orchestrate the annotation pipeline, ensuring smooth data flow from preprocessing to final annotation.
Quality Control and Review: Ensuring annotation quality is crucial for training accurate machine learning models. Annotation tools often offer functionalities for reviewing and validating annotations. They allow supervisors or experienced annotators to check and correct annotations, provide feedback, and maintain a consistent annotation standard across the dataset.
Automation and AI Assistance: To accelerate the annotation process, data annotation tools leverage automation techniques and AI assistance. For instance, they may provide pre-trained models for tasks like text classification or object recognition, which can be fine-tuned with user annotations. These tools can also suggest annotations or predict potential mistakes to guide annotators and improve efficiency.
What is MLOps ?
While data annotation tools play a crucial role in developing Machine Learning models, they are just one piece of the puzzle in the broader context of MLOps (Machine Learning Operations). MLOps encompasses the practices and tools for effectively managing the lifecycle of Machine Learning models, from development to deployment and monitoring. It effectively combines Machine Learning (ML) with the principles of DevOps to manage the end-to-end lifecycle of Machine Learning models.
In essence, MLOps is the process that keeps ML models running smoothly, from the initial data preparation stages to model deployment and beyond, allowing for a continuous feedback loop and improvement cycle. It's not just about building a model; it's about building a system that can scale, adapt, and maintain high performance in real-world scenarios.
The MLOps pipeline
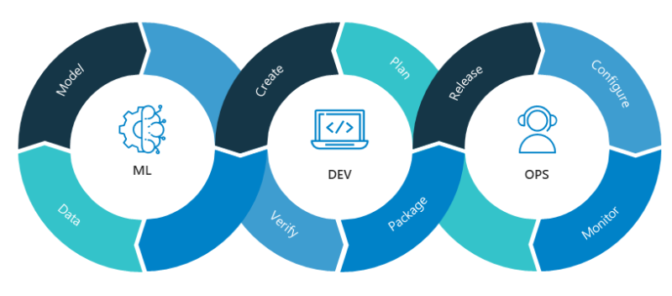
The MLOps pipeline is all about creating a structured approach to developing, deploying, and maintaining machine learning models in production. It’s an evolution of the traditional DevOps pipeline but tailored to the unique demands of Machine Learning. A typical MLOps pipeline involves the following stages:
Data preparation: The first step in the MLOps pipeline is data collection and annotation, the pipeline moves into the data preparation phase. This involves cleaning, transforming, and sometimes augmenting the data to make it suitable for training.
Model Development and Training: The next step is streamlining model development and training pipelines. This involves version control, experiment tracking, and reproducibility of results. Tools like TensorFlow, PyTorch, and scikit-learn provide libraries and frameworks for building and training models, while platforms like MLflow and DVC offer functionalities for managing the entire development lifecycle. This step also involves validating the ML model over validation datasets to keep track of what works and what doesn’t.
Deployment and Serving: After training a model, it needs to be deployed and served in production environments. A good MLOps pipeline ensures smooth deployment by providing tools for containerization, orchestration, and scalability. Platforms like Kubernetes and Docker enable the packaging and deployment of machine learning models as containers, while tools like TensorFlow Serving and Seldon Core simplify the serving process.
Monitoring and Maintenance: Once a model is deployed, it requires continuous monitoring to ensure its performance and reliability. It involves setting up continuous integration and continuous deployment (CI/CD) systems to automate the deployment process. A good MLOps pipeline should use monitoring tools to track model metrics, detect anomalies, and trigger alerts. Platforms like Prometheus and Grafana offer powerful monitoring capabilities, while tools like TensorBoard help visualize and analyze model performance.
The feedback loop: Finally there’s the feedback loop. Real-world data can expose weaknesses in your model that you didn’t catch during testing. The MLOps pipeline should be designed to capture this feedback and use it to improve the model. This could involve collecting new data, refining the annotations, or even revisiting the model architecture.
The future of MLOps and Data Annotation tools
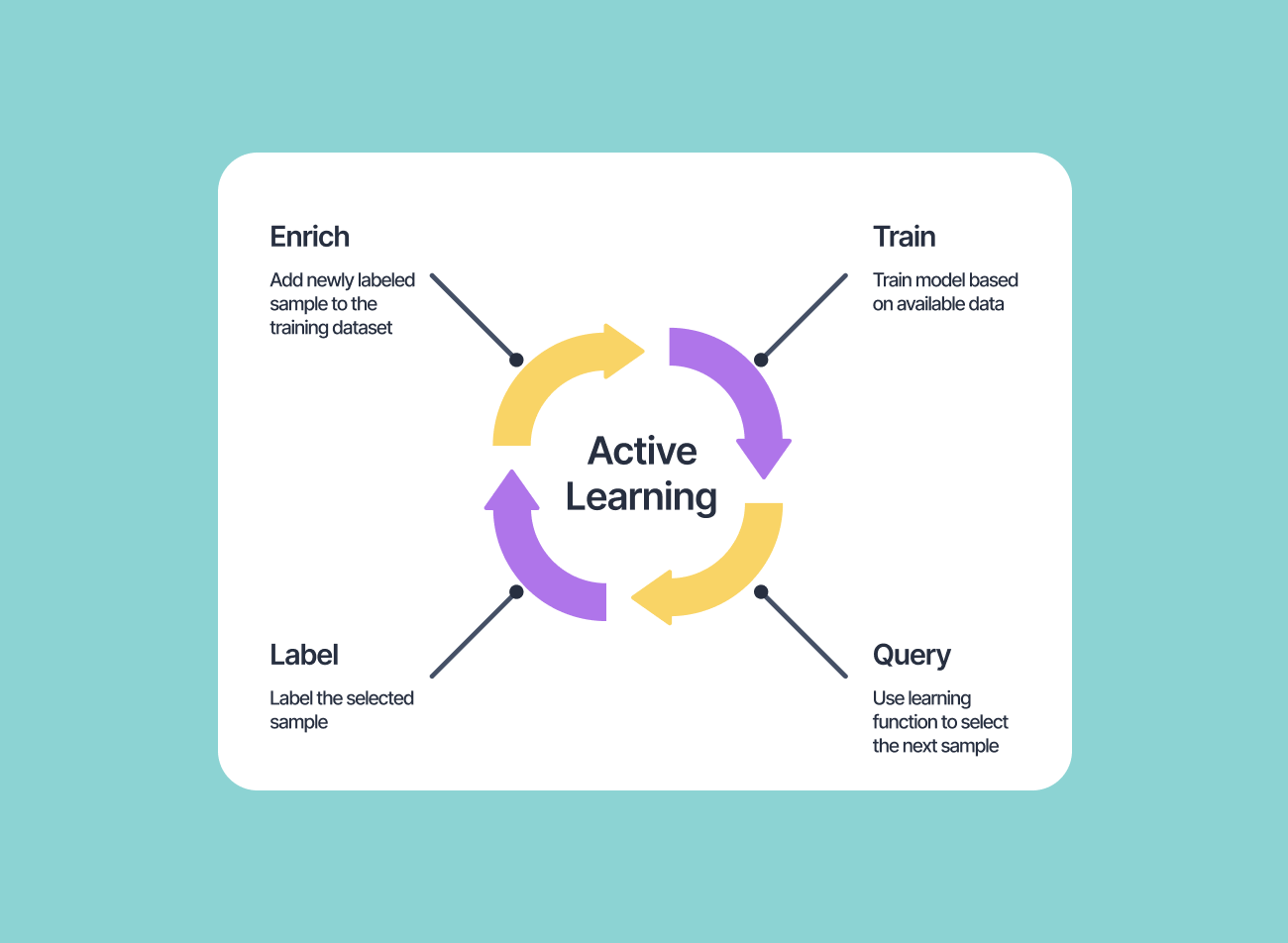
As machine learning continues to advance, the field of MLOps and data annotation tools will continue to evolve. Here are some key trends and future directions:
Active Learning and Semi-Supervised Learning: Active learning techniques aim to reduce annotation effort by automatically selecting the most informative data points for annotation. Data annotation tools will likely integrate active learning capabilities, allowing annotators to focus on challenging or uncertain samples. Semi-supervised learning approaches, which leverage a combination of labeled and unlabeled data, will also play a significant role in reducing annotation requirements. You can read about how Tesla uses Active Learning to judiciously upload new data scenarios in this article.
Explainability and Interpretability: As machine learning models are increasingly deployed in critical domains, the need for model explainability and interpretability becomes crucial. MLOps and data annotation tools will focus on incorporating techniques to generate explanations for model predictions and make them more interpret-able to humans. This will enable better trust and understanding of machine learning models.
Automated Data Labeling: Fully automating the data annotation process is an ongoing area of research. Data annotation tools will continue to explore techniques such as weak supervision, active learning, and transfer learning to reduce human annotation effort. By leveraging existing labeled data or pre-trained models, these tools aim to generate accurate annotations with minimal human intervention.
Conclusion
Data annotation tools have become indispensable in the development of machine learning models. They empower annotators with efficient and effective annotation capabilities, enhancing the quality and accuracy of labeled datasets. Meanwhile, MLOps provides a comprehensive framework for managing the entire lifecycle of machine learning models, ensuring smooth development, deployment, and monitoring. As the field progresses, we can expect data annotation tools and MLOps practices to evolve further, enabling the development of even more advanced and reliable machine learning models.