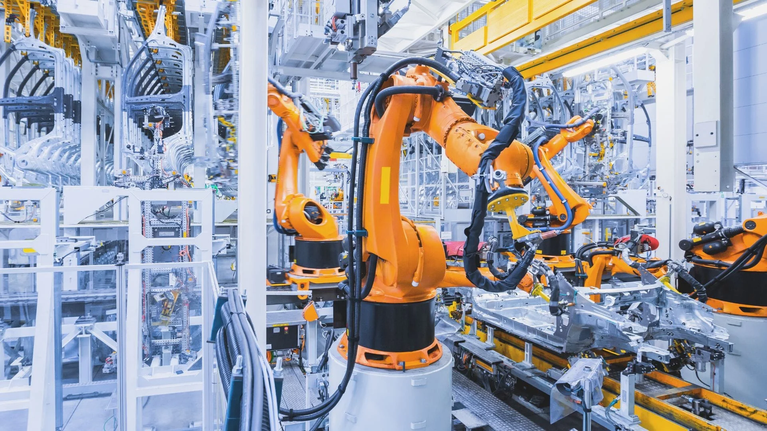
In the manufacturing industry, quality assurance is a critical aspect of the production process that ensures the final products meet the required standards and specifications. Traditionally, quality assurance and defect detection have been achieved through manual inspections and sampling, which can be time-consuming and error-prone. However, with the advent of artificial intelligence (AI), manufacturers can now leverage intelligent systems to improve the speed, accuracy, and efficiency of defect detection.
In this blog post, we will explore how AI is revolutionizing defect detection in manufacturing, discuss the various AI technologies being utilized, and examine the benefits and challenges of implementing AI-based quality assurance systems.
The Importance of Defect Detection in Manufacturing
Defect detection is a critical process in manufacturing that aims to identify and rectify any defects or imperfections in products before they reach the end consumer. Ensuring product quality and reliability is essential for several reasons:
- Customer Satisfaction: High-quality products lead to increased customer satisfaction and loyalty. Defects can result in customer complaints, returns, and loss of trust in the brand.
- Cost Reduction: Early detection of defects helps prevent costly recalls, rework, and wasted materials. By catching defects early in the production process, manufacturers can reduce production costs.
- Regulatory Compliance: Many industries have strict regulations and standards that require products to meet specific quality criteria. Non-compliance can result in legal issues, fines, and damage to the company's reputation.
- Competitive Advantage: Companies known for producing high-quality products have a competitive edge in the market. Defect detection plays a key role in maintaining quality standards and differentiating a company from its competitors.
How AI is Transforming Defect Detection
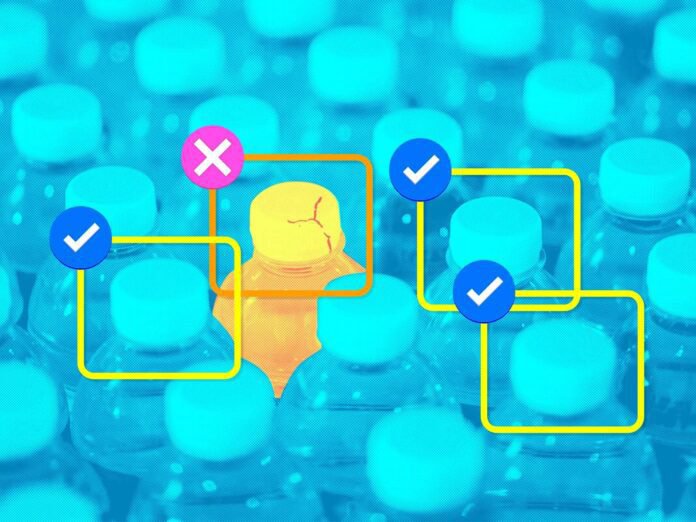
Artificial intelligence has the potential to revolutionize defect detection in manufacturing by providing intelligent and automated solutions that can analyze vast amounts of data and identify defects with high precision. Here are some key AI technologies being used in defect detection:
Computer Vision
Computer vision, a sub-field of AI, uses machine learning and deep learning algorithms to enable computers to interpret and analyze visual information. In manufacturing, computer vision can be applied to defect detection in the following ways:
- Image Analysis: AI-powered cameras can capture high-resolution images of products as they move along the production line. These images are then analyzed to detect any visual defects, such as scratches, cracks, or discoloration.
- Pattern Recognition: AI models can be trained to recognize specific patterns associated with defects. For example, an AI system can learn to identify defects in fabric based on patterns of color variation.
- Object Detection: AI can detect the presence or absence of specific features on a product, such as missing components or incorrect assembly.
Anomaly Detection
Anomaly detection is a technique that involves identifying unusual patterns or outliers in data that deviate from the norm. In manufacturing, anomaly detection can be applied to:
- Sensor Data Analysis: AI algorithms can analyze data from sensors placed throughout the production line to detect anomalies in machine performance or product quality.
- Statistical Modeling: AI can create statistical models of normal behavior and compare new data against these models to identify deviations that may indicate defects.
Natural Language Processing (NLP)
NLP, a branch of AI focused on understanding and processing human language, can be used in manufacturing for defect detection in the following ways:
- Textual Data Analysis: AI can analyze textual data such as inspection reports or customer feedback to identify trends or patterns related to defects.
- Voice Recognition: AI-powered voice recognition systems can be used for real-time voice commands to control inspection equipment or provide feedback on defects.
Predictive Maintenance
Predictive maintenance leverages AI to forecast potential equipment failures and schedule maintenance before defects occur. This approach can help manufacturers avoid production downtime and improve overall quality.
- Historical Data Analysis: AI algorithms can analyze historical data on equipment performance to predict when maintenance is needed.
- Real-Time Monitoring: AI can monitor equipment in real-time and identify early signs of failure, allowing for proactive maintenance.
Benefits of AI in Defect Detection
The integration of AI into defect detection processes in manufacturing offers numerous benefits, including:
Improved Accuracy
AI-powered defect detection systems can analyze large datasets and identify defects with high precision. This level of accuracy reduces the risk of false positives and false negatives, ensuring that only defective products are identified.
Increased Efficiency
AI systems can operate continuously without fatigue, unlike human inspectors. This allows for real-time inspection and reduces production delays caused by manual inspections.
Cost Savings
By identifying defects early in the production process, manufacturers can reduce material waste and rework costs. Additionally, predictive maintenance can help prevent costly equipment failures.
Enhanced Safety
AI systems can detect potential safety hazards in products or equipment, helping to prevent accidents and ensure a safer working environment.
Scalability
AI systems can easily scale to handle large volumes of data and adapt to different manufacturing environments, making them suitable for a wide range of industries and production lines.
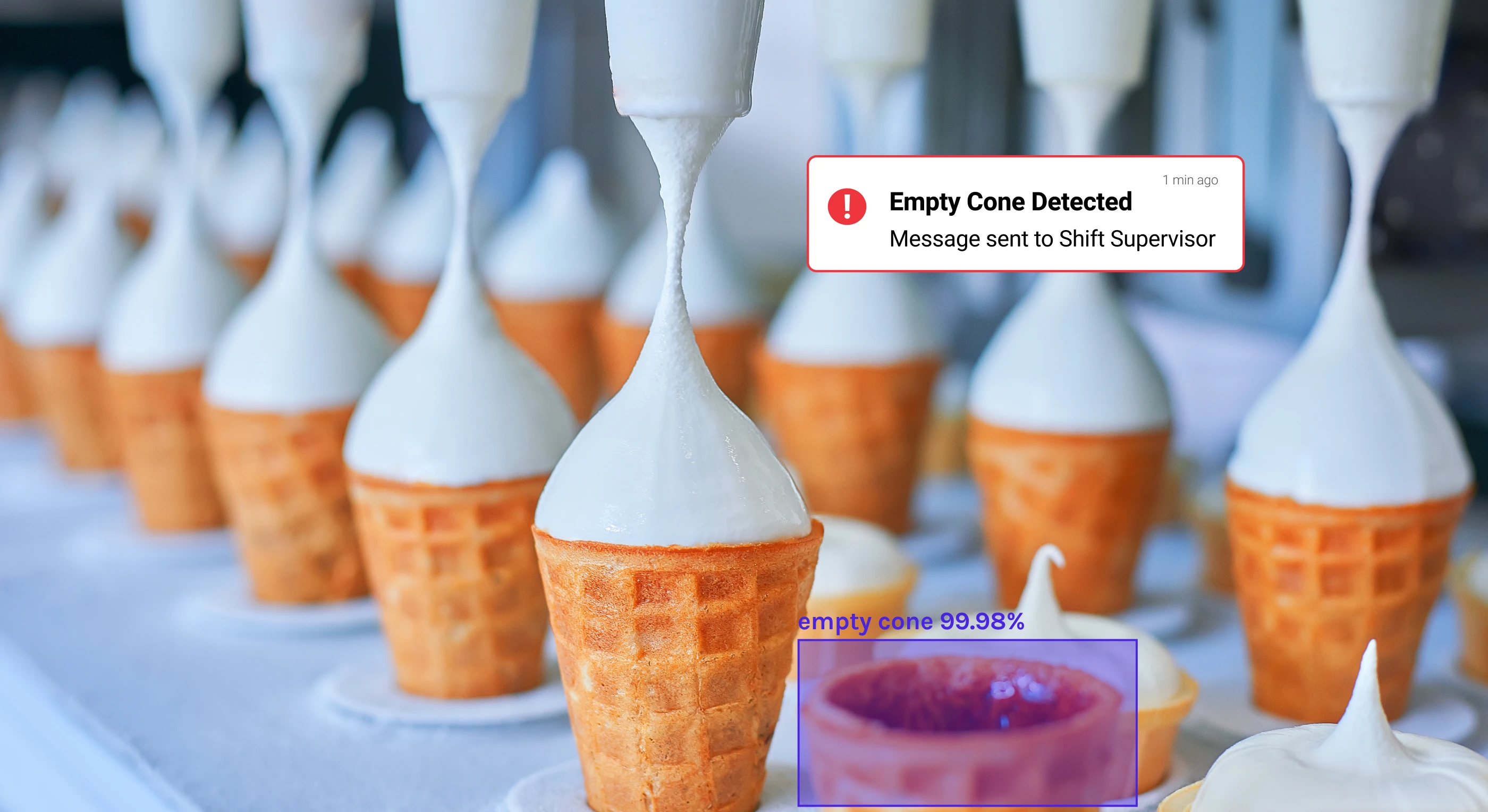
Challenges of Implementing AI in Defect Detection
While AI offers significant advantages for defect detection in manufacturing, there are also challenges associated with its implementation:
Quality and Availability
AI models require high-quality data to learn and make accurate predictions. In some cases, manufacturers may struggle to collect and maintain sufficient data for training AI models.
Integration with Existing Systems
Integrating AI-based defect detection systems with existing manufacturing processes and equipment can be complex and may require significant investments in infrastructure.
Interpretability
AI models can sometimes be difficult to interpret, making it challenging for manufacturers to understand how decisions are made and ensure transparency in quality assurance processes.
Workforce Training and Adaptation
Introducing AI into manufacturing may require training the workforce to work with new technologies and adapt to changes in the production process.
Cost of Implementation
While AI can lead to long-term cost savings, the initial investment in AI technology and infrastructure can be substantial.
Future Trends and Developments
The use of AI in defect detection in manufacturing is expected to continue growing as technology advances. Some potential future trends include:
Edge Computing
Edge computing brings AI processing closer to the data source, reducing latency and improving real-time defect detection. This approach is particularly beneficial for fast-paced production environments.
AI-Powered Robots
AI-powered robots can be used for automated inspection and defect detection. These robots can operate autonomously and adapt to changing production conditions.
Advanced Sensors and IoT Integration
The integration of AI with advanced sensors and the Internet of Things (IoT) can provide manufacturers with real-time data for continuous monitoring and defect detection.
AI as a Service
AI as a service (AIaaS) allows manufacturers to access AI-powered defect detection solutions through cloud-based platforms, reducing the need for in-house AI expertise and infrastructure.
Explainable AI
Explainable AI focuses on making AI models more interpretable and transparent. This can help manufacturers understand how decisions are made and ensure compliance with industry standards and regulations.
Conclusion
AI is transforming defect detection in manufacturing by providing intelligent and automated solutions that improve the accuracy, efficiency, and cost-effectiveness of quality assurance processes. While there are challenges to implementing AI in manufacturing, the potential benefits far outweigh the hurdles. As technology continues to advance, manufacturers can expect to see even more innovative and impactful applications of AI in defect detection.
By leveraging AI, manufacturers can enhance product quality, reduce costs, improve customer satisfaction, and maintain a competitive edge in the market. As AI becomes more accessible and integrated into manufacturing processes, the industry will continue to evolve, leading to new opportunities for growth and innovation.