Point clouds are a crucial element in the fields of 3D modeling and spatial data analysis. They are used in a variety of applications, from autonomous driving to archaeology, providing detailed and accurate representations of physical environments. In this blog, we will explore what point clouds are, delve into Lidar point clouds, and discuss their significance and applications in various industries.
What is a lidar sensor?
Lidar has revolutionized the way we gather and analyze data about our surroundings. Lidar sensors use highly focused and precise beams of light to measure distances from objects and create 3D maps of the environment. By emitting short pulses of light and measuring the time it takes for them to bounce back, Lidar systems can create accurate point cloud representations of the surroundings.
A brief history of Lidar
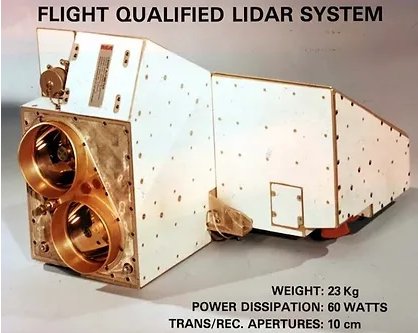
The basic idea behind Lidar was first introduced in the 1960s, but it wasn't until the 1980s that it became a viable technology for remote sensing applications. The initial use of LiDAR was primarily for analyzing terrain and creating topographic maps. However, with advancements in laser and sensor technology, LiDAR evolved to capture more detailed and accurate data, leading to its widespread use in various industries such as urban planning, forestry and archaeology. The advent of solid-state lidar in last decade, which is more compact and cost-effective, has made Lidars ubiquitous in the autonomous vehicle industry.
What is a Point Cloud?
A point cloud is a collection of data points defined in a three-dimensional space. Each point represents a specific location in the environment and contains information about its coordinates (X, Y, Z). These points collectively form a "cloud" that can represent the external surface of an object or a landscape in great detail.
Key characteristics of Point Clouds
- 3D Coordinates: Each point is defined by its X, Y, and Z coordinates in a three-dimensional space.
- Density: The density of points can vary, with higher densities providing more detailed representations.
- Color and Intensity: Some point clouds also include additional data such as color (RGB values) and intensity, adding more context to the spatial information.
How Are Point Clouds Generated?
Point clouds can be generated using various technologies, including:
Laser Scanners
Laser scanners emit laser beams to measure distances to surfaces, generating dense, accurate point clouds. They work by sending out laser pulses and measuring the time it takes for the reflections to return to the scanner.
Photogrammetry
Photogrammetry is the process of producing 3D pointclouds from 2D images taken from different angles. By analyzing multiple overlapping photographs of an object or scene, photogrammetry software can reconstruct the 3D geometry of the subject.
LiDAR (Light Detection and Ranging)
A remote sensing method that uses light in the form of a pulsed laser to measure variable distances to the Earth. Lidars produce the most accurate point clouds and can cover longer distances compared to the other methods of collecting 3D data.
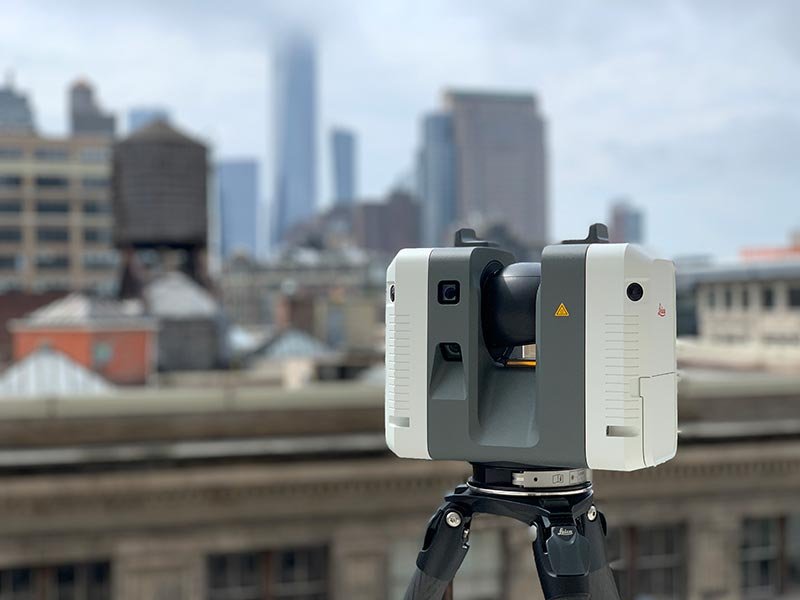
Lidar vs point cloud
Lidar, as described above is a type of remote sensing technology that uses laser pulses to measure the distance to an object or surface. The data collected by a Lidar is typically in the form of a point cloud, which is a collection of individual data points in a three-dimensional coordinate system. So, in simple terms, Lidar is the technology used to scan the environment and collect the data, while a point cloud is the result or output of the scan. In other words, while Lidar refers to the technology itself, point cloud refers to the visual representation of the data collected by Lidar.
Key Characteristics of Lidar Point Clouds:
- High Precision: Lidar sensors can produce extremely accurate and detailed point clouds, with high spatial resolution.
- Speed: Lidar systems can quickly capture large areas, making them ideal for applications that require rapid data acquisition over large geographical areas like forestry and surveying.
- Versatility: Lidar can be used in various environments, including terrestrial, aerial, and underwater applications.
Significance of point density in Lidar point clouds
Point density is a crucial factor in point cloud LiDAR data collection and analysis. It refers to the number of points per unit volume captured by the LiDAR sensor. A rough parallel to point cloud density can be drawn with image resolution or the number of pixels in an image. The more the resolution, the more detailed the image will be. The same can be applied to point clouds. A higher point density means more points are captured, resulting in a more accurate and precise 3D model of the terrain. This is particularly important for applications such as topographic mapping, infrastructure planning, and disaster management, where accurate data is crucial for decision making. Point density also affects the quality and resolution of the point cloud, making it an important consideration for LiDAR data processing.
Lidar point cloud data formats
There are a variety of lidar point cloud file formats in use today. Here are some of the most widely used formats:
LAS format
LAS is widely used for saving aerial point clouds captured by Lidars mounted on drones or airplanes. These scans provide a bird's-eye view of large geographical areas and are extensively used in forest management and surveying. Learn more about LAS here.
PCD format
PCD is the most widely used file format for lidar point clouds. PCD files can be in ASCII or Binary formats - while ASCII files can be easily processed and viewed, binary files are smaller in size which can be a huge advantage for large point cloud files.
PLY format
PLY is another format used to save Lidar point clouds. It is similar to PCD in terms of the structure of the files - the header contains information about the contents of the file, and the body contains the actual data points.
You can learn more about the different point cloud file formats and how to process themin this article.
Colorizing lidar point clouds
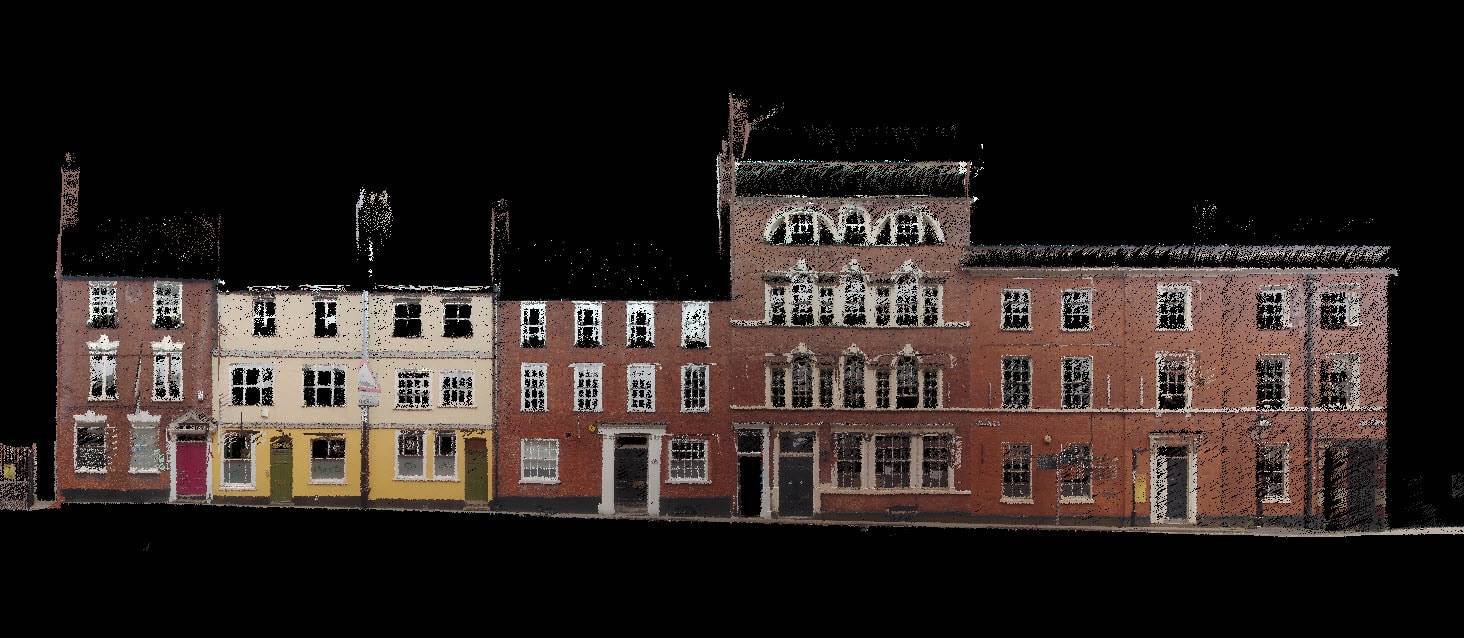
Lidars can only capture the location of points on the surface of an object. As a result, raw Lidar point clouds are not colored. However, adding RGB colors provides an important layer of information, making it easier to process and analyze point clouds.
One way to colorize Lidar point clouds from standalone camera imagery is to use a process called image registration. This involves aligning a point cloud with the camera images using common features such as edges or corners. Once the registration is complete, the color information from the camera images can be projected onto the corresponding points in the point cloud.
There is another way to produce colorized point clouds if the sensors (Lidar and cameras) are properly calibrated and capture features from the environment at the same time. In this case, the position of the sensors relative to each other combined with intrinsic camera parameters can be used to locate points captured by lidar on the camera images, providing color to the point clouds.
Multi-sensor fusion of Lidar and cameras
Multi-sensor fusion is an advanced technology that combines data from different sensors to provide a more accurate and comprehensive understanding of a particular environment or situation. By fusing data from multiple sources such as cameras, radars, and lidars, this technology can overcome the limitations of individual sensors and provide a more holistic view. This has numerous applications in various industries, from self-driving cars to military surveillance systems. You can learn more about Lidar sensor fusion here.
Advantages of lidar point clouds over traditional sensors
There are numerous advantages of using point cloud lidar technology over traditional sensors.
- Lidar can capture high-resolution 3D data, providing a more detailed and accurate representation of the environment. This allows for precise measurements and better understanding of the surrounding, compared to cameras.
- Lidar is also not affected by lighting conditions, making it a reliable option for both day and night use.
- Compared with other 3D scanning techniques like photogrammetry, Lidar point clouds can detect objects at longer distances, making them ideal for applications such as autonomous driving and aerial mapping.
Challenges with Lidar point clouds
While lidar point clouds offer numerous advantages, they also present several challenges:
- High cost
One of the main challenges faced in point cloud LiDAR technology is the high cost associated with acquiring and processing data. LiDAR sensors are expensive and require specialized equipment, making it difficult for smaller companies or organizations to access this technology - Data Volume
Lidar point clouds can contain millions of points, resulting in large data volumes that require significant storage and processing power. - Data Noise
Lidar point clouds can include noise and outliers, which need to be filtered out to ensure accurate representations. - Integration with other sensor data
Integrating point clouds with other data sources, such as 2D maps or imagery, can be complex and requires advanced processing techniques.
Applications of Lidar Point Clouds
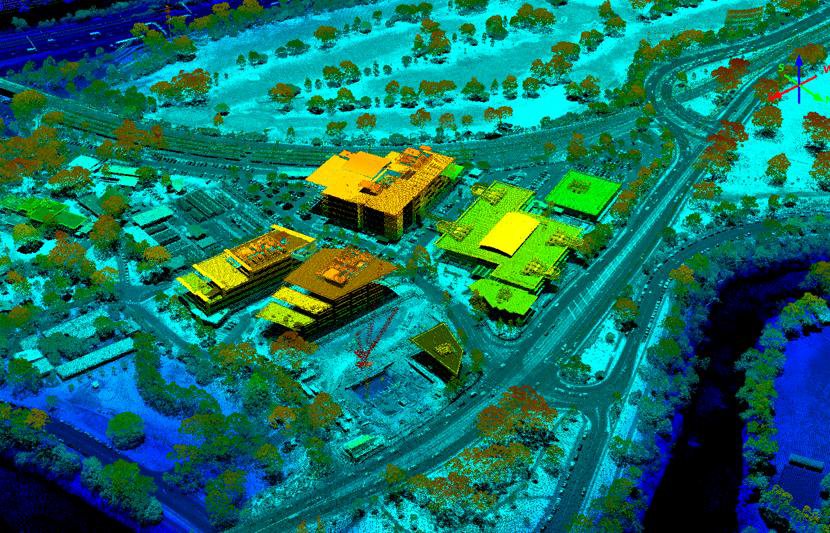
Lidar Point clouds have a wide range of applications across various industries, providing detailed and accurate 3D representations that are invaluable for analysis and decision-making.
Autonomous Driving
- Environment Mapping: Lidar Point clouds are used to create detailed maps of the environment, helping autonomous vehicles navigate safely.
- Obstacle Detection: Lidar Point clouds enable the detection and identification of obstacles in the vehicle's path.
Construction and Architecture
- Building Information Modeling (BIM): Point clouds are used for BIM to create accurate 3D models of buildings and structures, facilitating design and construction processes.
- Site Surveying: Point clouds provide detailed topographical data, aiding in site planning and construction management.
Forestry and Environmental Monitoring
- Forest Inventory: Lidar point clouds are used to assess forest biomass, tree height, and canopy structure, aiding in forest management.
- Terrain Analysis: Lidar Point clouds help in analyzing terrain features, monitoring erosion, and managing natural resources.
Urban Planning and Infrastructure
- City Modeling: Point clouds are used to create 3D models of urban areas, facilitating urban planning and infrastructure development.
- Utility Management: Point clouds assist in mapping and managing utilities such as power lines, pipelines, and transportation networks.
Archaeology
Lidar point clouds are used to create detailed records of archaeological sites and historical structures, preserving them digitally for future study. Lidars are also helpful to discover archaeological sites buried beneath the surface.
Parting thoughts
Lidar point clouds are a powerful tool for capturing and representing three-dimensional environments with high accuracy and detail providing invaluable information for a wide range of applications, from autonomous driving and urban planning to archaeology and environmental monitoring. As Lidar technology continues to advance, the capabilities and applications of Lidar point clouds will expand, driving innovation and enhancing our ability to understand and interact with the world around us.